Adversary Instantiation: Lower Bounds for Differentially Private Machine Learning
2021 IEEE Symposium on Security and Privacy (SP)(2021)
摘要
Differentially private (DP) machine learning allows us to train models on private data while limiting data leakage. DP formalizes this data leakage through a cryptographic game, where an adversary must predict if a model was trained on a dataset D, or a dataset D′ that differs in just one example. If observing the training algorithm does not meaningfully increase the adversary's odds of successful...
更多查看译文
关键词
Training,Deep learning,Privacy,Differential privacy,Upper bound,Toxicology,Games
AI 理解论文
溯源树
样例
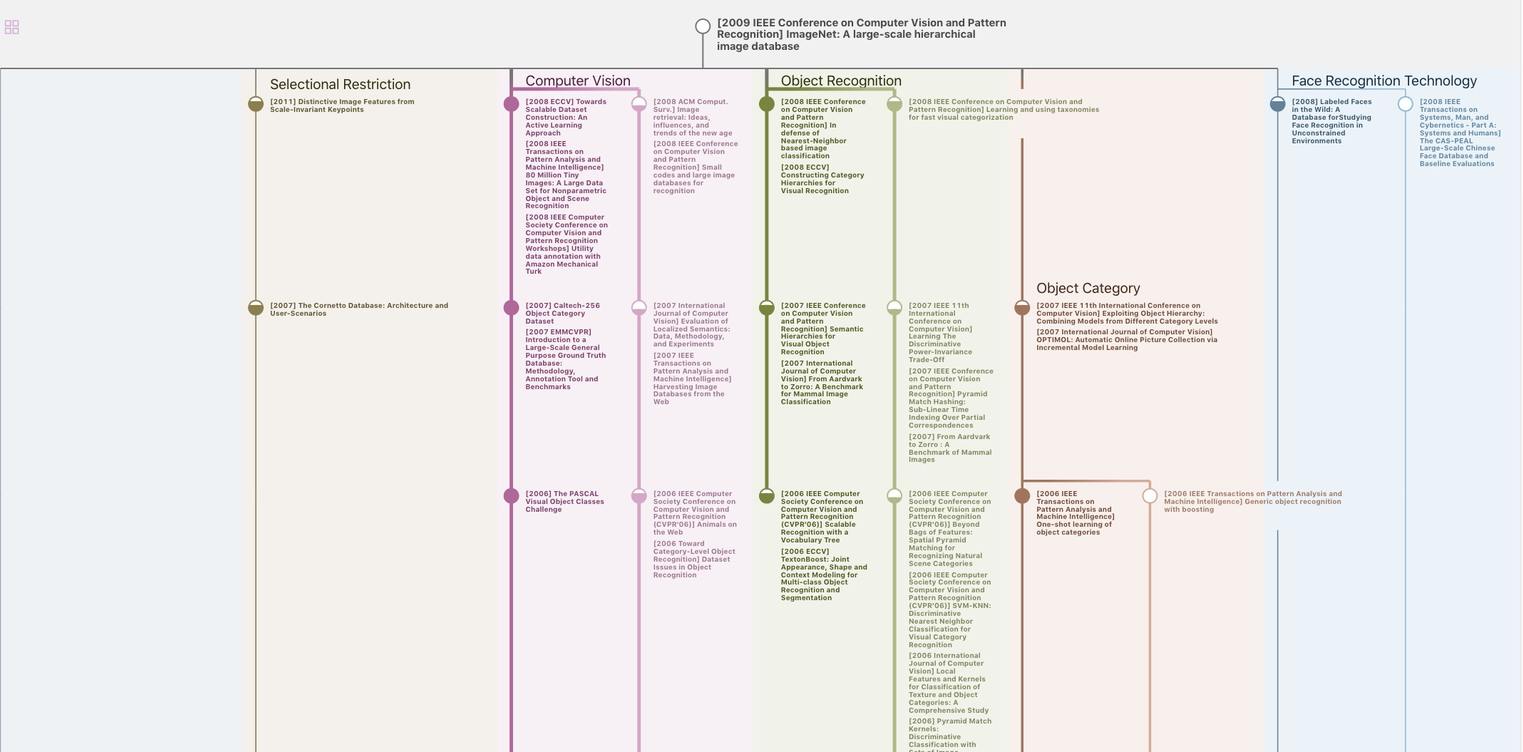
生成溯源树,研究论文发展脉络
Chat Paper
正在生成论文摘要