Detection Of Weather Events In Optical Satellite Data Using Deep Convolutional Neural Networks
REMOTE SENSING LETTERS(2021)
摘要
Early detection and forecast of extreme weather events such as tropical cyclones and wildfires are becoming increasingly crucial for mitigating their catastrophic damages. Remotely-sensed satellite data enable us to detect and assimilate these events into weather models to improve their global-scale forecasts. Despite the significant advances in satellite imagery and image processing techniques, weather data assimilation methods currently employ conventional approaches that can be slow and inaccurate. This letter aims to bridge this gap by utilizing deep convolutional neural networks (CNN) to accurately and rapidly detect weather patterns in satellite images. InceptionV3, ResNet50, VGG16, and VGG19, as four state-of-the-art CNN models, are used to classify 9081 optical satellite images into five different weather events of convective cell clouds, tropical cyclones, convective roll clouds, wildfires, and dust storms. All these models obtained a satisfactory average accuracy of more than 80%. Among the CNNs, VGG-Nets were found to have difficulties in distinguishing roll convection from cell convection patterns. Overall, InceptionV3 was the best model by achieving an average accuracy of 92% in detecting such weather systems. The letter demonstrates the strength of these CNN techniques for weather pattern recognition that can be instrumental for atmospheric science research and weather/climate models.
更多查看译文
AI 理解论文
溯源树
样例
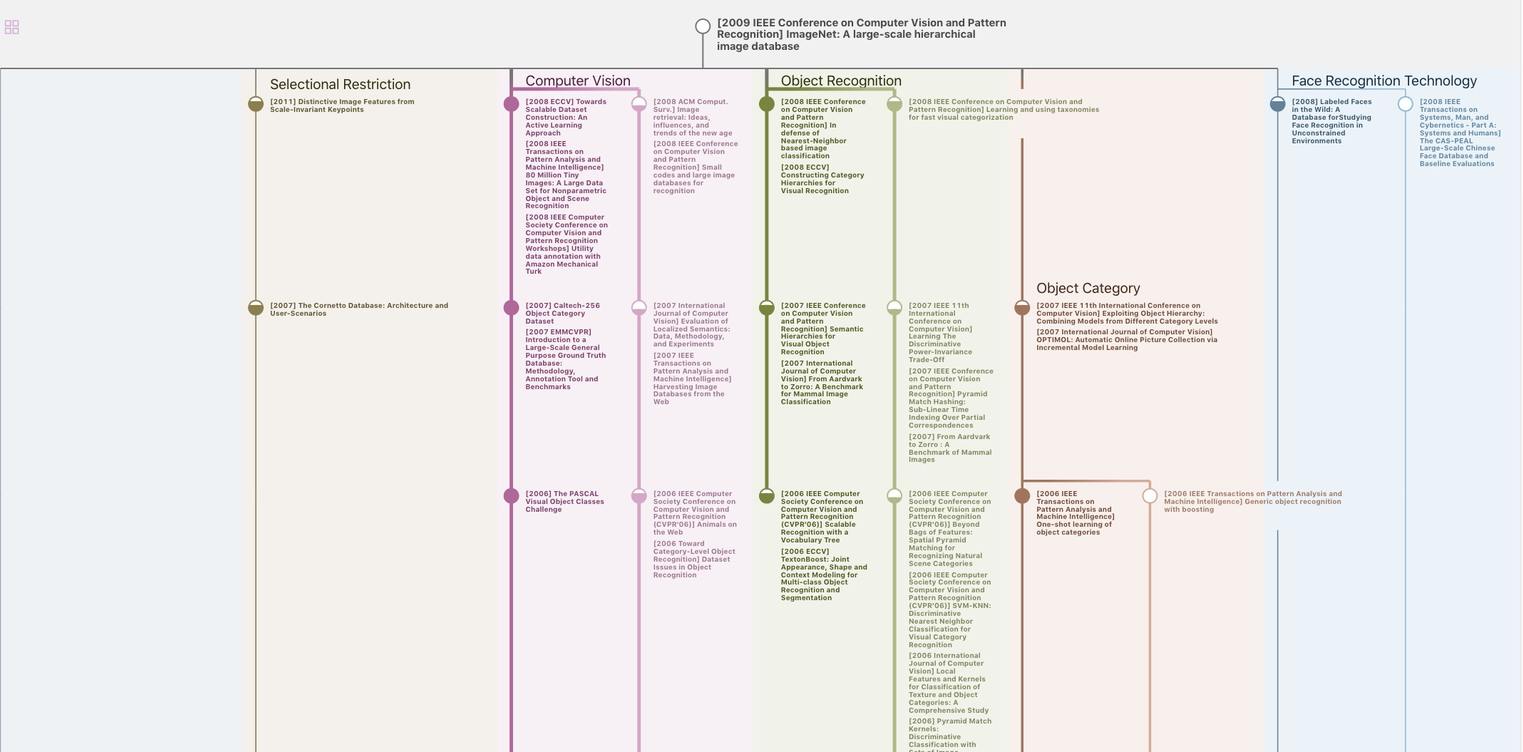
生成溯源树,研究论文发展脉络
Chat Paper
正在生成论文摘要