A Generative Model for Partial Label Learning.
ICME(2021)
摘要
Partial label (PL) learning tackles the problem where each training instance is associated with a set of candidate labels, among which only one is the true label. In this paper, we propose a novel generative model PL-CGAN, which tackles the partial label learning problem with Conditional Generative Adversarial Nets (CGAN). Specially, PL-CGAN introduces a teacher model to refine a more reliable soft label vector of each training instance by iteratively ensembling the current learned prediction network with the formal one in an online manner. Besides, it adopts a MixUp data augmentation scheme to prevent the prediction network from overfitting to the noisy labels. In addition, it deploys a CGAN to generate training instances with its corresponding label vectors, which form a feature alignment based on consistency cost to enhance it’s label refinement capacity. Extensive experiments are conducted on synthesized and real-world partial label learning datasets, while the proposed approach demonstrates the state-of-the-art performance for partial label learning.
更多查看译文
关键词
learned prediction network,data augmentation scheme,real-world partial label learning datasets,label refinement capacity,noisy labels,reliable soft label vector,conditional generative adversarial nets,partial label learning problem,generative model PL-CGAN,candidate labels
AI 理解论文
溯源树
样例
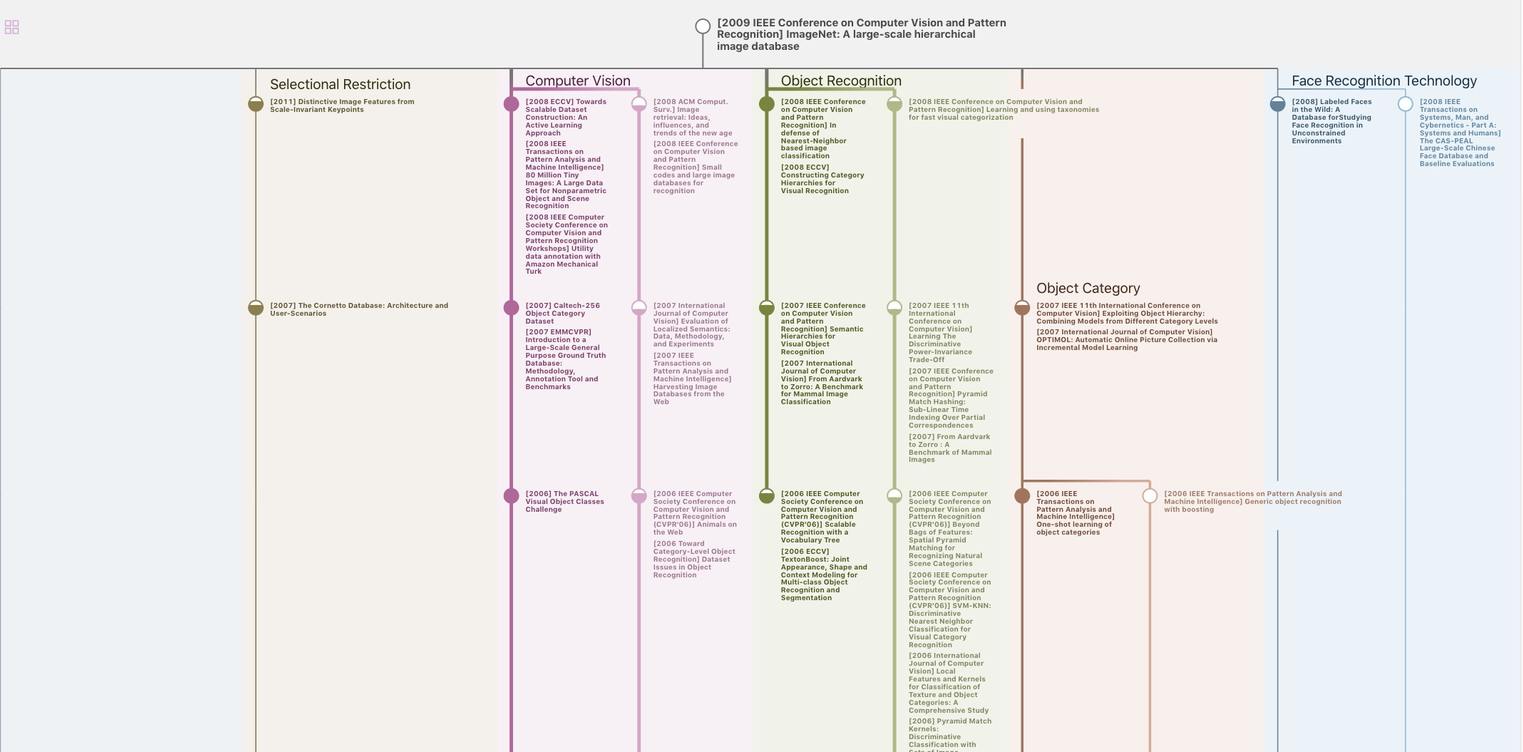
生成溯源树,研究论文发展脉络
Chat Paper
正在生成论文摘要