Correction to: Fast data-driven model reduction for nonlinear dynamical systems
arxiv(2023)
摘要
We present a fast method for nonlinear data-driven model reduction of dynamical systems onto their slowest nonresonant spectral submanifolds (SSMs). While the recently proposed reduced-order modeling method SSMLearn uses implicit optimization to fit a spectral submanifold to data and reduce the dynamics to a normal form, here, we reformulate these tasks as explicit problems under certain simplifying assumptions. In addition, we provide a novel method for timelag selection when delay-embedding signals from multimodal systems. We show that our alternative approach to data-driven SSM construction yields accurate and sparse rigorous models for essentially nonlinear (or non-linearizable ) dynamics on both numerical and experimental datasets. Aside from a major reduction in complexity, our new method allows an increase in the training data dimensionality by several orders of magnitude. This promises to extend data-driven, SSM-based modeling to problems with hundreds of thousands of degrees of freedom.
更多查看译文
关键词
Invariant manifolds,Reduced-order modeling,Spectral submanifolds,Normal forms,Machine learning
AI 理解论文
溯源树
样例
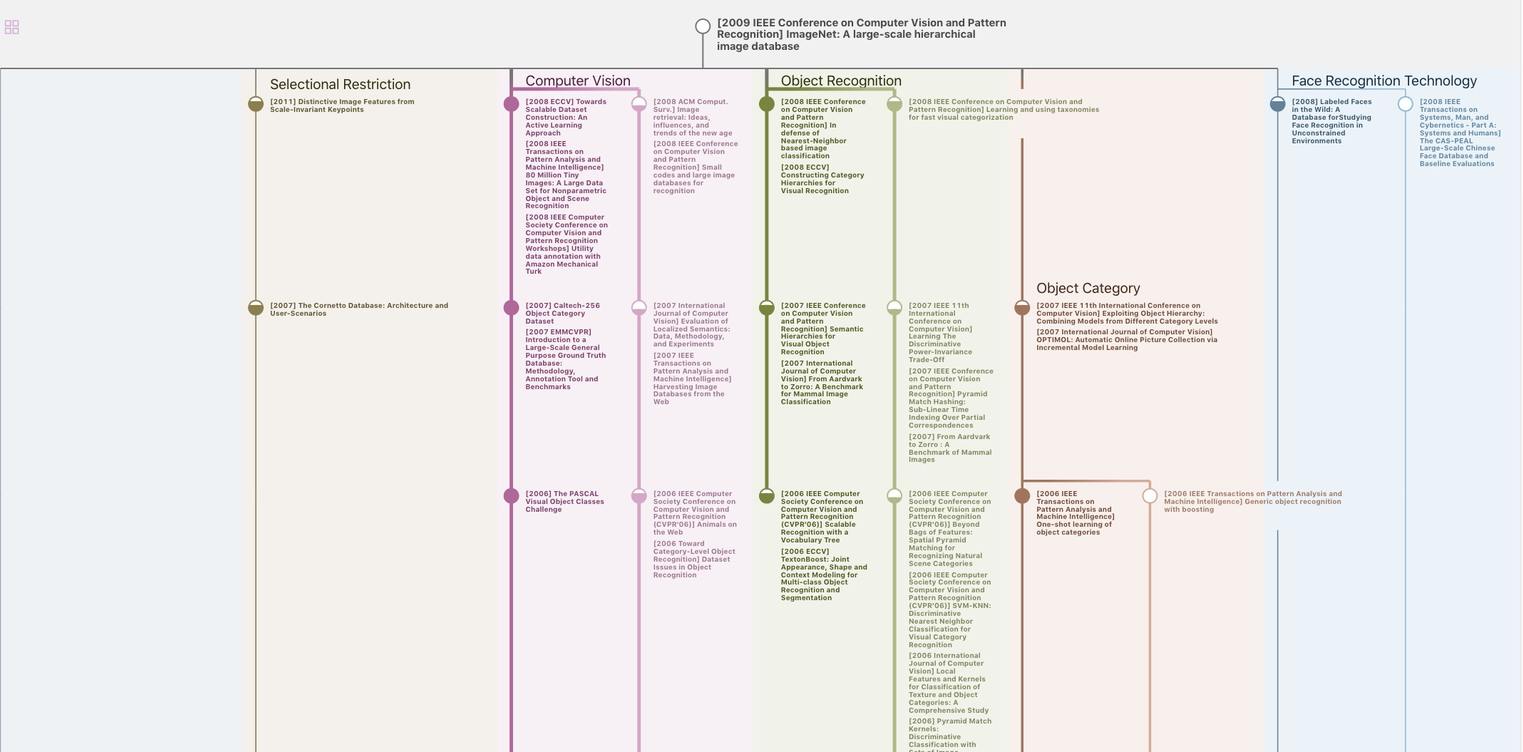
生成溯源树,研究论文发展脉络
Chat Paper
正在生成论文摘要