Wave Glider Speed Model for Real-time Motion Planning
OCEANS 2021: San Diego – Porto(2021)
摘要
The present study examines the use of four distinct machine learning frameworks to predict and characterize the speed response of the SV3 Wave Glider on timescales relevant to local motion planning. Models are trained using onboard measurements of incident waves and near-surface currents. The research draws on a 71-day dataset collected during two deployments conducted between Oct-Dec 2020 and Apr-Jun 2021 off the coast of Southern California. The observations span a wide range of environmental conditions, with significant wave heights and near surface flow speeds ranging up to 6 m and 0.8 m/s, respectively. Predictions generated by the best-performing models showed good quantitative agreement when evaluated with multiple independent datasets, indicating that the selected features and models generalize well. A Gaussian process model produced the highest skill ($r^{2}=0.87$) and lowest generalization errors (RMSE = 7cm/s), although marginal differences were found when compared against the ensemble tree and neural network model outputs. Analysis of permutation-based feature importance estimates indicates that along-track currents and short-period $(T\lt 6 {\mathrm s})$ waves provide the bulk of the contribution to the speed model predictions, suggesting that the vehicle’s speed response is largely dominated by wind-seas and near surface currents.
更多查看译文
关键词
Wave Glider, machine learning, USV, path planning
AI 理解论文
溯源树
样例
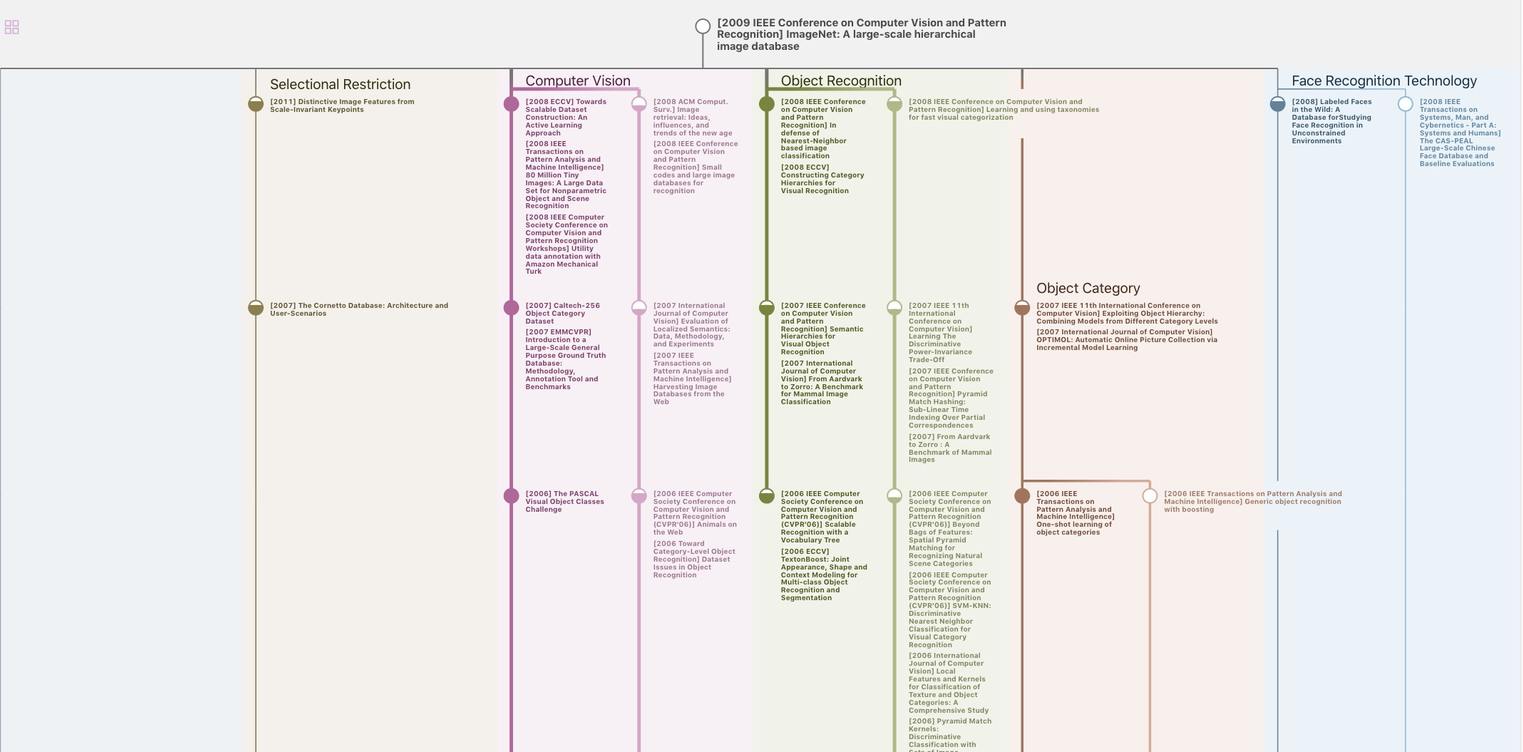
生成溯源树,研究论文发展脉络
Chat Paper
正在生成论文摘要