Variational Transfer Learning using Cross-Domain Latent Modulation
arxiv(2022)
摘要
To successfully apply trained neural network models to new domains, powerful transfer learning solutions are essential. We propose to introduce a novel cross-domain latent modulation mechanism to a variational autoencoder framework so as to achieve effective transfer learning. Our key idea is to procure deep representations from one data domain and use it to influence the reparameterization of the latent variable of another domain. Specifically, deep representations of the source and target domains are first extracted by a unified inference model and aligned by employing gradient reversal. The learned deep representations are then cross-modulated to the latent encoding of the alternative domain, where consistency constraints are also applied. In the empirical validation that includes a number of transfer learning benchmark tasks for unsupervised domain adaptation and image-to-image translation, our model demonstrates competitive performance, which is also supported by evidence obtained from visualization.
更多查看译文
关键词
variational transfer learning,modulation,cross-domain
AI 理解论文
溯源树
样例
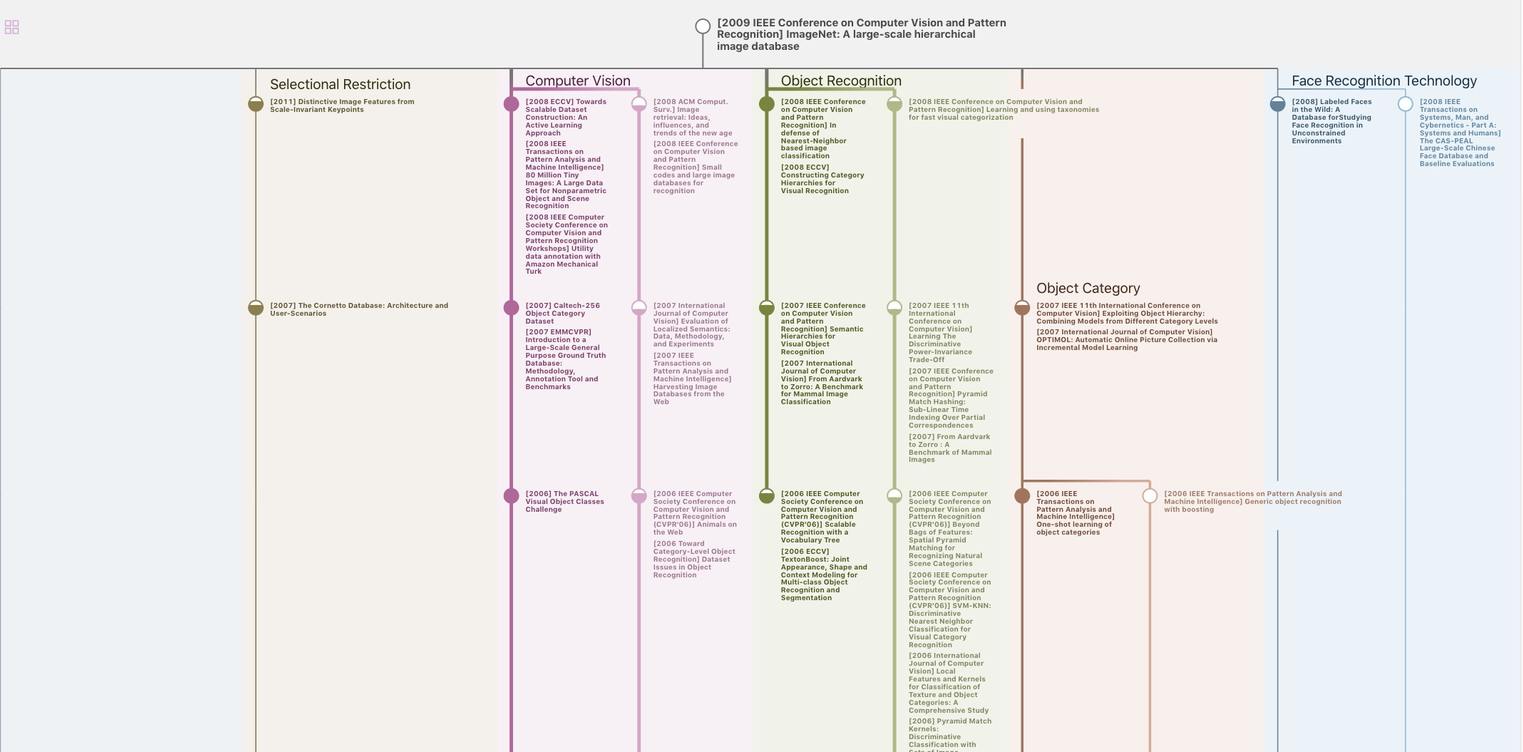
生成溯源树,研究论文发展脉络
Chat Paper
正在生成论文摘要