Cuvier's beaked whale foraging dives identified via machine learning using depth and triaxial acceleration
MARINE ECOLOGY PROGRESS SERIES(2022)
摘要
Knowledge of Cuvier's beaked whale Ziphius cavirostris behavior has expanded through the utilization of animal-borne tags. However, many tag types do not record sound- thus preventing echolocation click detections to identify foraging- or have short deployments that sample a limited range of behaviors. As the quantity of such non-acoustic tag data grows, so too does the need for robust methods of detecting foraging from non-acoustic data. We used 692 dives from 5 sound-recording tags on Cuvier's beaked whales in southern California, USA, to develop extreme gradient boosting tree models to detect foraging based on 1 Hz depth and 16 Hz triaxial acceleration data. We performed repeated 10-fold cross validation using classification accuracy to tune 500 models with randomly partitioned training and testing datasets. An average of 99.9 and 99.2% of training and testing dataset dives, respectively, were correctly classified across the 500 models. Dives without associated sound recordings (n = 2069 from 7 whales including 4 nonacoustic tags) were classified via a model that maximized training information using dive depth and duration, ascent and descent rates, bottom-phase average vertical speed, and roll circular variance during dive descents and bottom phases. Of all long, deep dives (conventionally assumed to include foraging), 2.4% were classified as non-foraging dives, while 0.3% of short, shallow dives were classified as foraging dives. Results confirm that conventional depth and/or duration classifiers provide reasonable estimates of longer-term foraging patterns. However, additional variables previously listed enhance foraging detections for unusual dives (notably nonforaging deep dives) for southern California Cuvier's beaked whales.
更多查看译文
关键词
Cuvier's beaked whale, Ziphius cavirostris, Foraging, Diving, Machine learning, Tagging
AI 理解论文
溯源树
样例
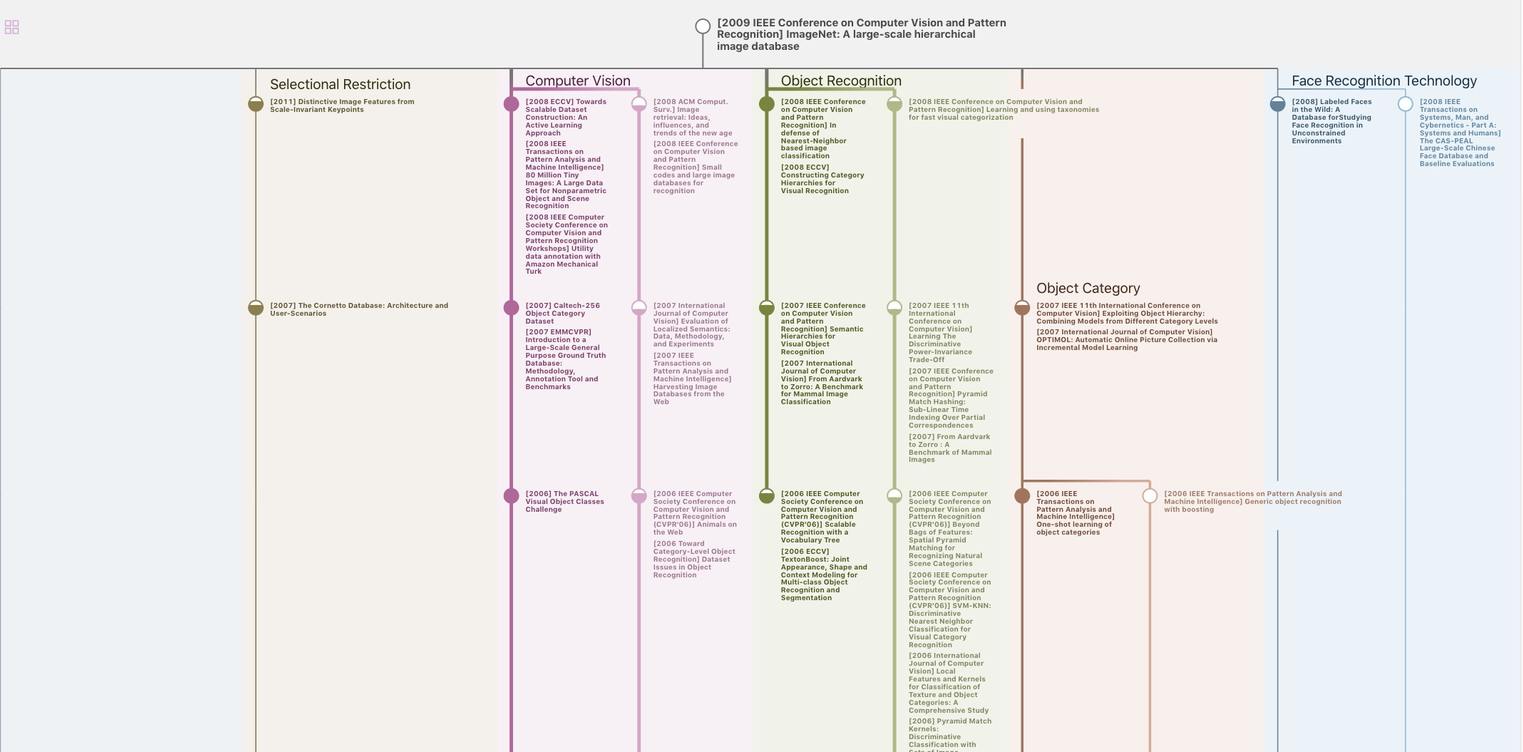
生成溯源树,研究论文发展脉络
Chat Paper
正在生成论文摘要