Stochastic dynamical wake modeling for wind farms
arxiv(2022)
摘要
Low-fidelity analytical models of turbine wakes have traditionally been used for wind farm planning, performance evaluation, and demonstrating the utility of advanced control algorithms in increasing the annual energy production. In practice, however, it remains challenging to correctly estimate the flow and achieve significant performance gains using conventional low-fidelity models. This is due to the over-simplified static nature of wake predictions from models that are agnostic to the effects of atmospheric boundary layer turbulence and the complex aerodynamic interactions among wind turbines. To improve the predictive capability of low-fidelity models while remaining amenable to control design, we offer a stochastic dynamical modeling framework for capturing the effect of atmospheric turbulence on the thrust force and power generation as determined by the actuator disk concept. In this approach, we use stochastically forced linear models of the turbulent velocity field to augment the analytically computed wake velocity and achieve consistency with higher-fidelity models in capturing power and thrust force measurements. The power-spectral densities of our stochastic models are identified via convex optimization to ensure consistency with partially available velocity statistics or power and thrust force measurements while preserving model parsimony. We demonstrate the utility of our approach in capturing turbulence intensity variations behind wind turbines and estimating thrust force and power signals generated by large-eddy simulations of the flow over a cascade of turbines. Our results provide insight into the significance of sparse field measurements in recovering the statistical signature of wind farm turbulence using stochastic linear models.
更多查看译文
AI 理解论文
溯源树
样例
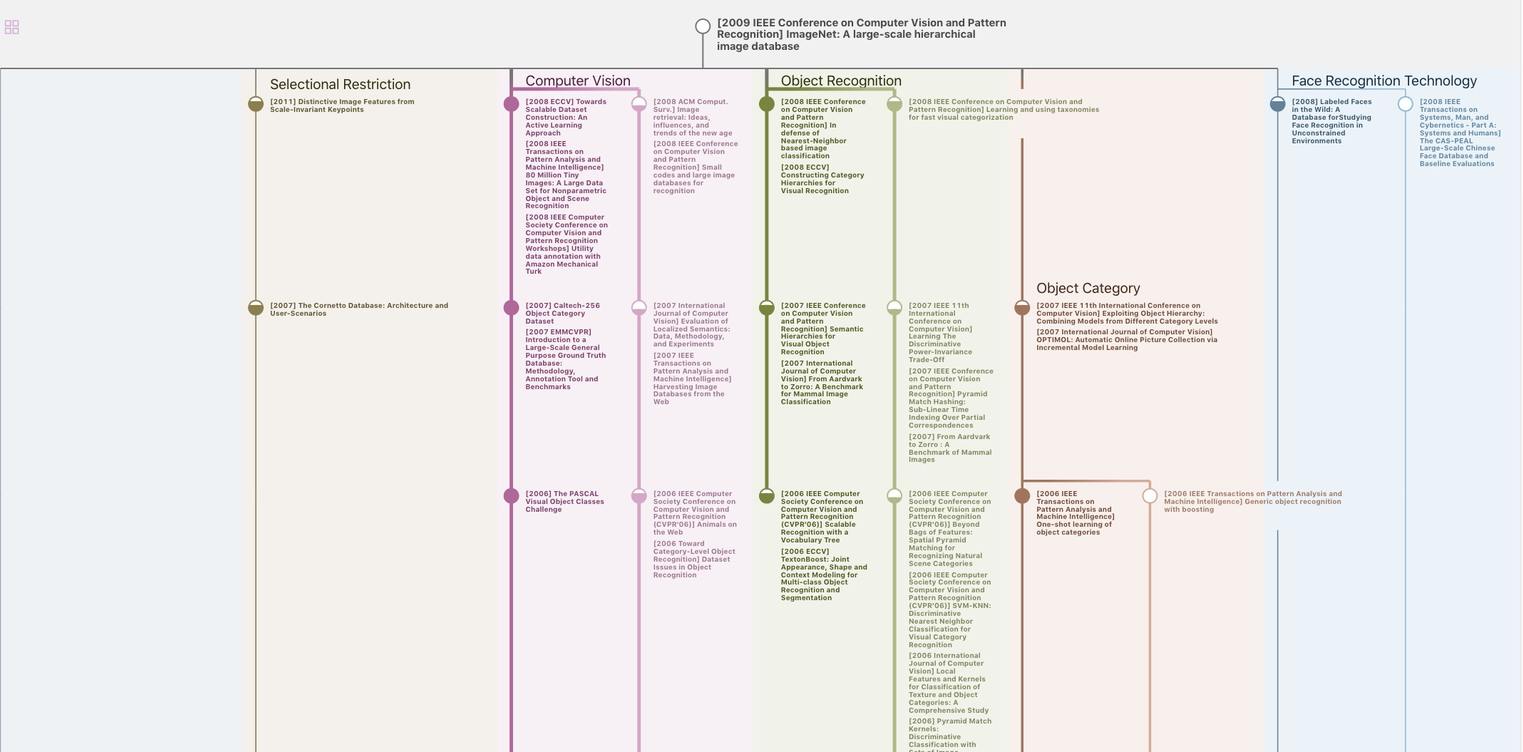
生成溯源树,研究论文发展脉络
Chat Paper
正在生成论文摘要