Frugal Hyperspectral Imaging via Low Rank Tensor Reconstruction
OCEAN SENSING AND MONITORING XIV(2022)
摘要
We develop a remote hyperspectral (HS) imaging work flow that relays spectral and spatial information of a scene via a minimal amount of encoded samples along with a robust data reconstruction scheme. To fully exploit the redundant and multidimensional structure of HS images, we adopt the canonical polyadic (CP) decomposition of multiway tensors. This approach represents our HS cube in a compressive manner while being naturally suitable for the linear mixing model, commonly used by practitioners to analyze the spectral content of each pixel. Under this low CP rank model we achieve frugal HS sensing by attenuating and encoding the incoming spectrum, thereby faithfully capturing the information with few measurements relative to its ambient dimensions. To further reduce the complexity of HS data, we apply image segmentation techniques to our encoded observations. By clustering the pixels into groups of endmembers with similar structure, we obtain a set of simplified data cubes each well approximated by a low CP rank tensor. To decode the measurements, we apply CP alternating least squares to each set of clustered pixels and combine the outputs to obtain our final HS image. We present several numerical experiments on synthetic and real HS data with various levels of input noise. We demonstrate that the approach outperforms state of the art methods, achieving noise attenuation while reducing the amount of collected data by a factor of 1/14.
更多查看译文
关键词
Hyperspectral imaging, canonical polyadic tensor decomposition, compressive sensing, remote sensing
AI 理解论文
溯源树
样例
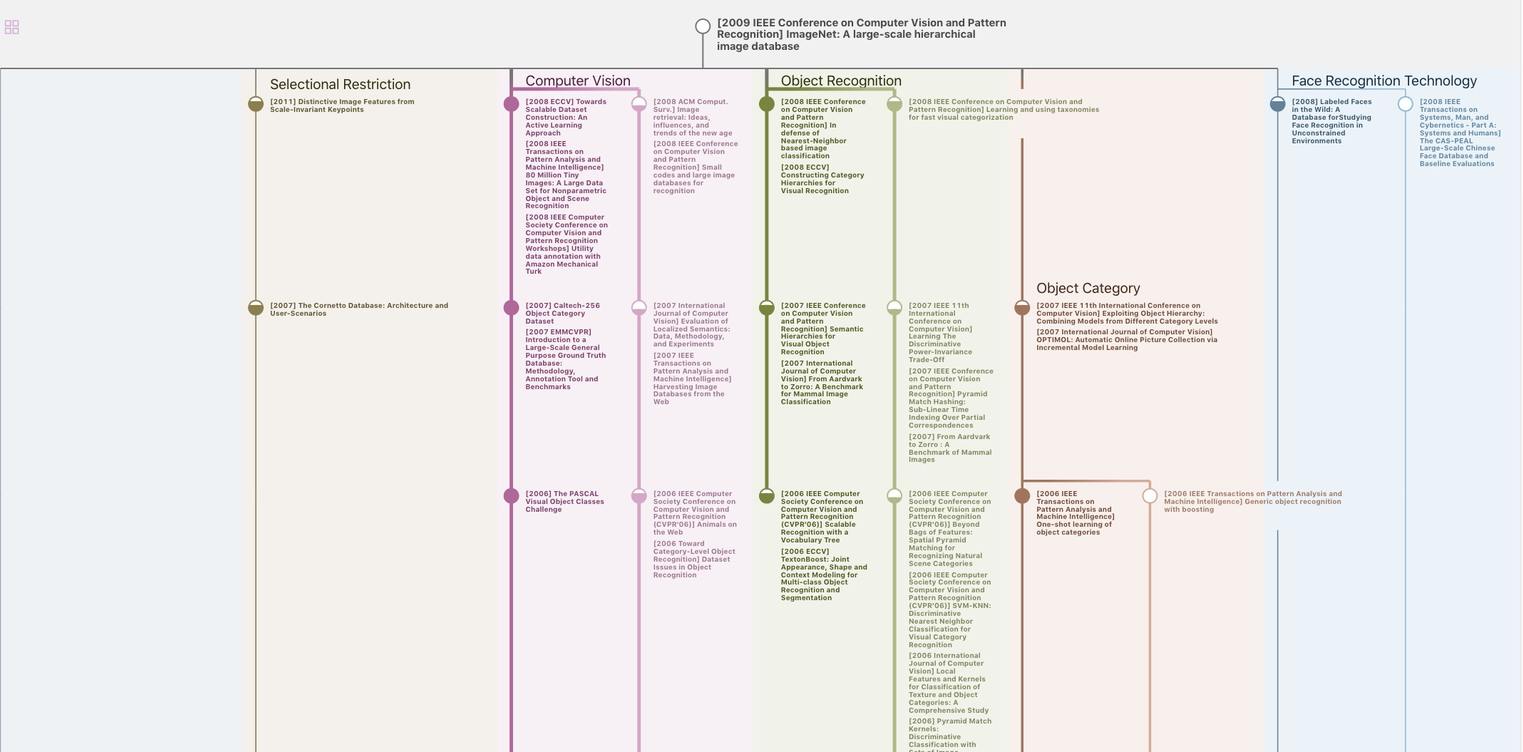
生成溯源树,研究论文发展脉络
Chat Paper
正在生成论文摘要