Storm Surge Forecast Using an Encoder-Decoder Recurrent Neural Network Model
Journal of Marine Science and Engineering(2022)
摘要
This study presents an encoder-decoder neural network model to forecast storm surges on the US North Atlantic Coast. The proposed multivariate time-series forecast model consists of two long short-term memory (LSTM) models. The first LSTM model encodes the input sequence, including storm position, central pressure, and the radius of the maximum winds to an internal state. The second LSTM model decodes the internal state to forecast the storm surge water level and velocity. The neural network model was developed based on a storm surge dataset generated by the North Atlantic Comprehensive Coastal Study using a physics-based storm surge model. The neural network model was trained to predict storm surges at three forecast lead times ranging from 3 h to 12 h by learning the correlation between the past storm conditions and future storm hazards. The results show that the computationally efficient neural network model can forecast a storm in a fraction of one second. The neural network model not only forecasts peak surges, but also predicts the time-series profile of a storm. Furthermore, the model is highly versatile, and it can forecast storm surges generated by different sizes and strengths of bypassing and landfalling storms. Overall, this work demonstrates the success of data-driven approaches to improve coastal hazard research.
更多查看译文
关键词
storm surge,Atlantic hurricanes,multivariate forecast,recurrent neural network,encoder-decoder model,long short-term memory model
AI 理解论文
溯源树
样例
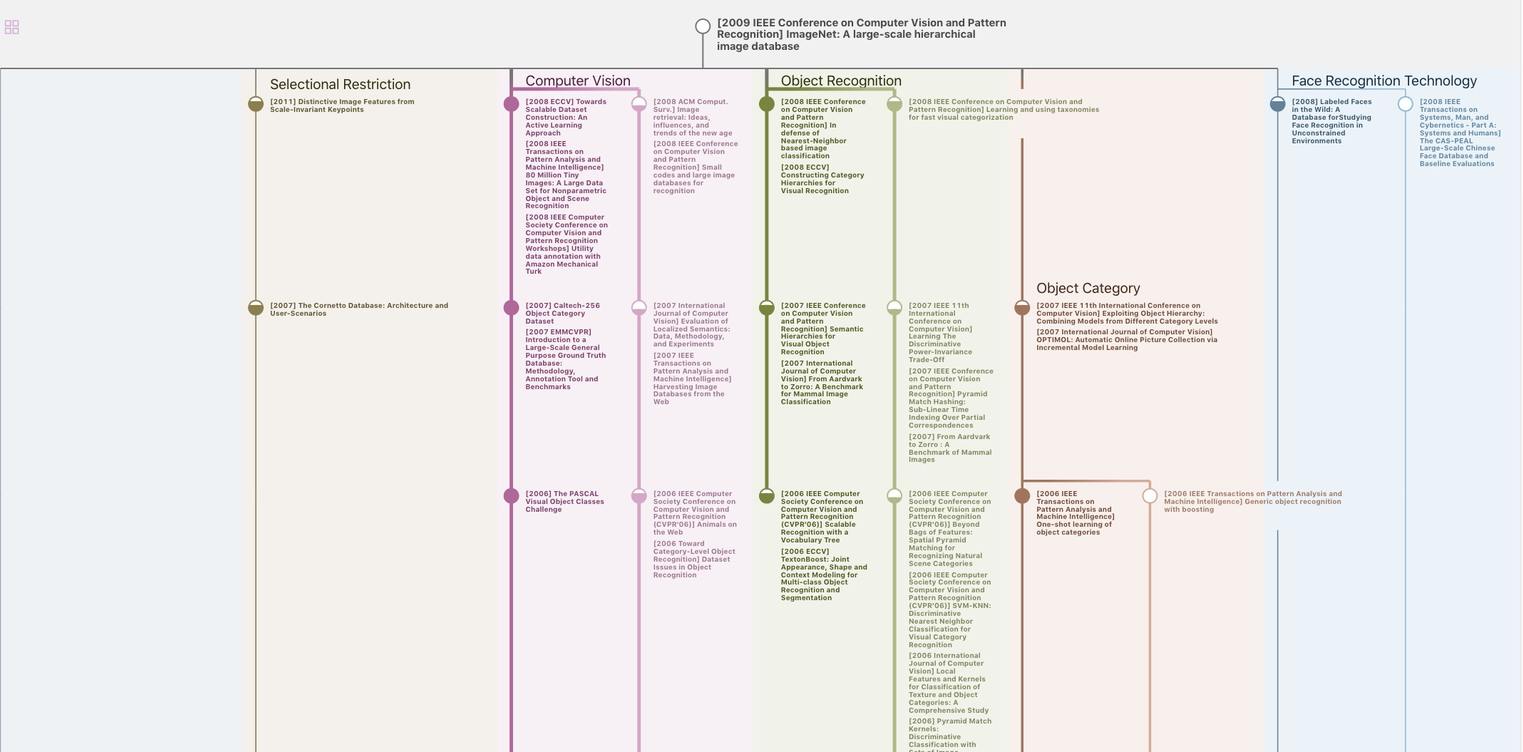
生成溯源树,研究论文发展脉络
Chat Paper
正在生成论文摘要