Predictions of photophysical properties of phosphorescent platinum(II) complexes based on ensemble machine learning approach
JOURNAL OF COMPUTATIONAL CHEMISTRY(2024)
摘要
Cyclometalated Pt(II) complexes are popular phosphorescent emitters with color-tunable emissions. To render their practical applications as organic light-emitting diodes emitters, it is required to develop Pt(II) complexes with high radiative decay rate constant and photoluminescence (PL) quantum yield. Here, a general protocol is developed for accurate predictions of emission wavelength, radiative decay rate constant, and PL quantum yield based on the combination of first-principles quantum mechanical method, machine learning, and experimental calibration. A new dataset concerning phosphorescent Pt(II) emitters is constructed, with more than 200 samples collected from the literature. Features containing pertinent electronic properties of the complexes are chosen and ensemble learning models combined with stacking-based approaches exhibit the best performance, where the values of squared correlation coefficients are 0.96, 0.81, and 0.67 for the predictions of emission wavelength, PL quantum yield and radiative decay rate constant, respectively. The accuracy of the protocol is further confirmed using 24 recently reported Pt(II) complexes, which demonstrates its reliability for a broad palette of Pt(II) emitters.
更多查看译文
关键词
DFT,machine learning,OLED,phosphorescent emitters,photophysical properties
AI 理解论文
溯源树
样例
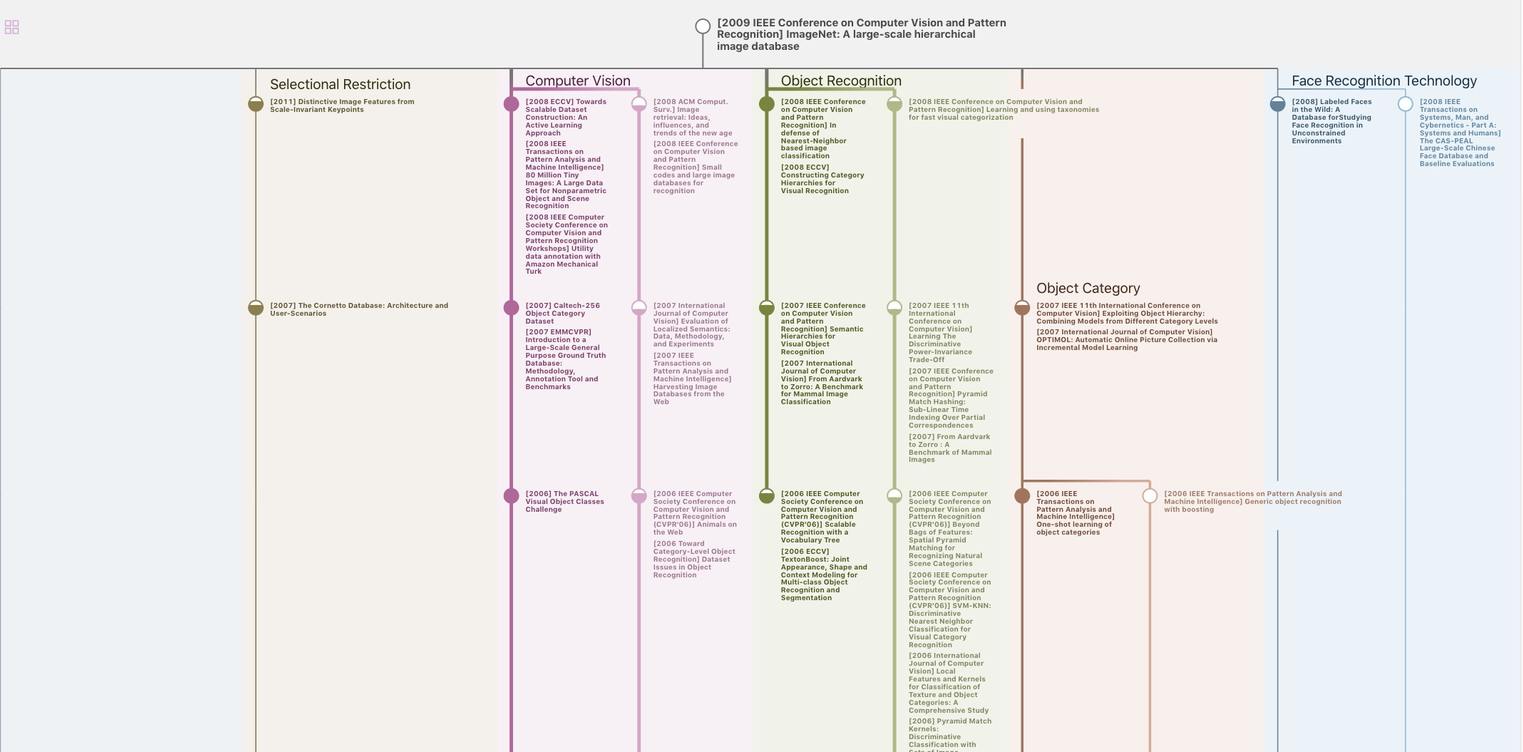
生成溯源树,研究论文发展脉络
Chat Paper
正在生成论文摘要