Partial Label Unsupervised Domain Adaptation with Class-Prototype Alignment
ICLR 2023(2023)
摘要
Partial label learning (PLL) tackles the problem where each instance is associated with a set of candidate labels, only one of which is the ground-truth. Most existing PLL approaches assume that both the training and test sets share the identical data distribution. However, this assumption does not hold in many real-world scenarios where the training and test data come from different distributions. In this paper, we formalize this learning scenario as a new problem called partial label unsupervised domain adaptation (PLUDA). To address this challenging PLUDA problem, we propose a novel Prototype Alignment based PLUDA method named PAPLUDA, which dynamically refines the pseudo-labels of instances from both the source and target domains by consulting the outputs of a teacher-student model in a moving-average manner, and bridges the domain discrepancy through inter- domain class prototype alignment. In addition, a teacher-student model based contrastive regularization is also deployed to enhance the prediction stability and hence improve the class prototypes in both domains for PLUDA. Comprehensive experimental results demonstrate that PAPLUDA achieves the state-of-the-art performance on the widely used benchmark datasets.
更多查看译文
关键词
Partial label learning,label noise,domain adaptation
AI 理解论文
溯源树
样例
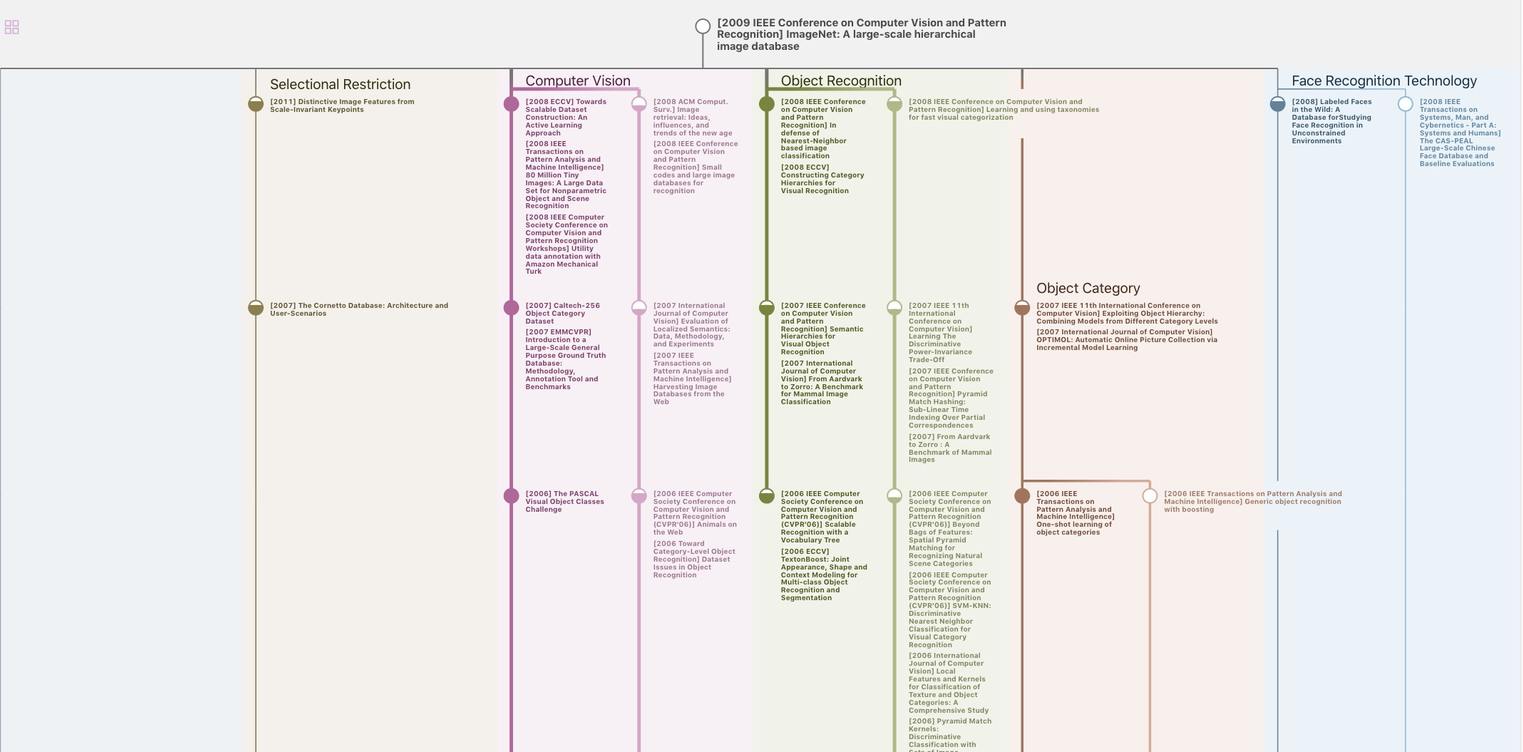
生成溯源树,研究论文发展脉络
Chat Paper
正在生成论文摘要