Optimization Applications as Quantum Performance Benchmarks
arxiv(2023)
摘要
Combinatorial optimization is anticipated to be one of the primary use cases for quantum computation in the coming years. The Quantum Approximate Optimization Algorithm (QAOA) and Quantum Annealing (QA) have the potential to demonstrate significant run-time performance benefits over current state-of-the-art solutions. Using existing methods for characterizing classical optimization algorithms, we analyze solution quality obtained by solving Max-Cut problems using a quantum annealing device and gate-model quantum simulators and devices. This is used to guide the development of an advanced benchmarking framework for quantum computers designed to evaluate the trade-off between run-time execution performance and the solution quality for iterative hybrid quantum-classical applications. The framework generates performance profiles through effective visualizations that show performance progression as a function of time for various problem sizes and illustrates algorithm limitations uncovered by the benchmarking approach. The framework is an enhancement to the existing open-source QED-C Application-Oriented Benchmark suite and can connect to the open-source analysis libraries. The suite can be executed on various quantum simulators and quantum hardware systems.
更多查看译文
关键词
quantum performance benchmarks,optimization
AI 理解论文
溯源树
样例
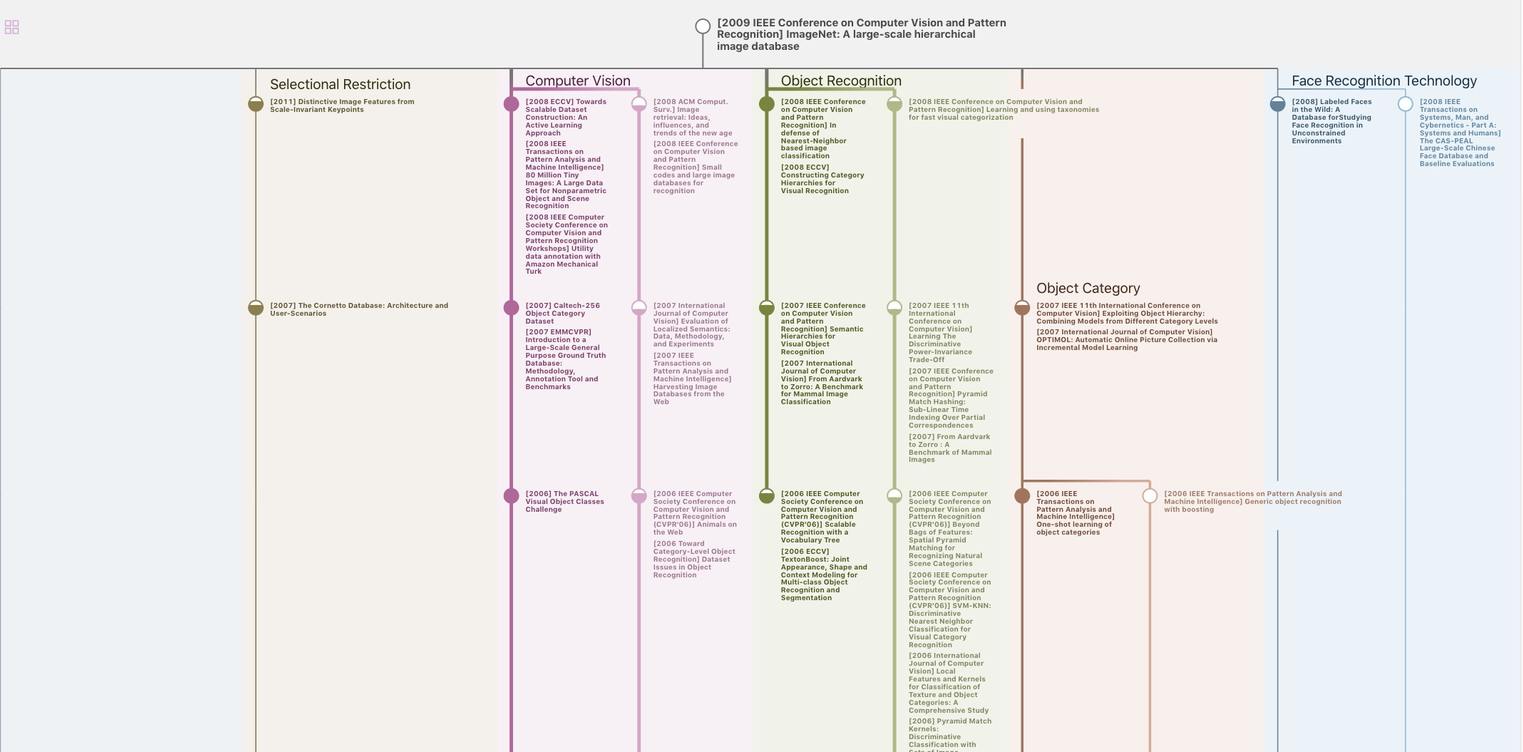
生成溯源树,研究论文发展脉络
Chat Paper
正在生成论文摘要