Transfer Learning for On-Orbit Ship Segmentation.
ECCV Workshops (1)(2022)
摘要
With the adoption of edge AI processors for space, on-orbit inference on EO data has become a possibility. This enables a range of new applications for space-based EO systems. Since the development of on-orbit AI applications requires rarely available raw data, training of these AI networks remains a challenge. To address this issue, we investigate the effects of varying two key image parameters between training and testing data on a ship segmentation network: Ground Sampling Distance and band misalignment magnitude. Our results show that for both parameters the network exhibits degraded performance if these parameters differ in testing data with respect to training data. We show that this performance drop can be mitigated with appropriate data augmentation. By preparing models at the training stage for the appropriate feature space, the need for additional computational resources on-board for e.g. image scaling or band-alignment of camera data can be mitigated.
更多查看译文
关键词
segmentation,transfer,learning,on-orbit
AI 理解论文
溯源树
样例
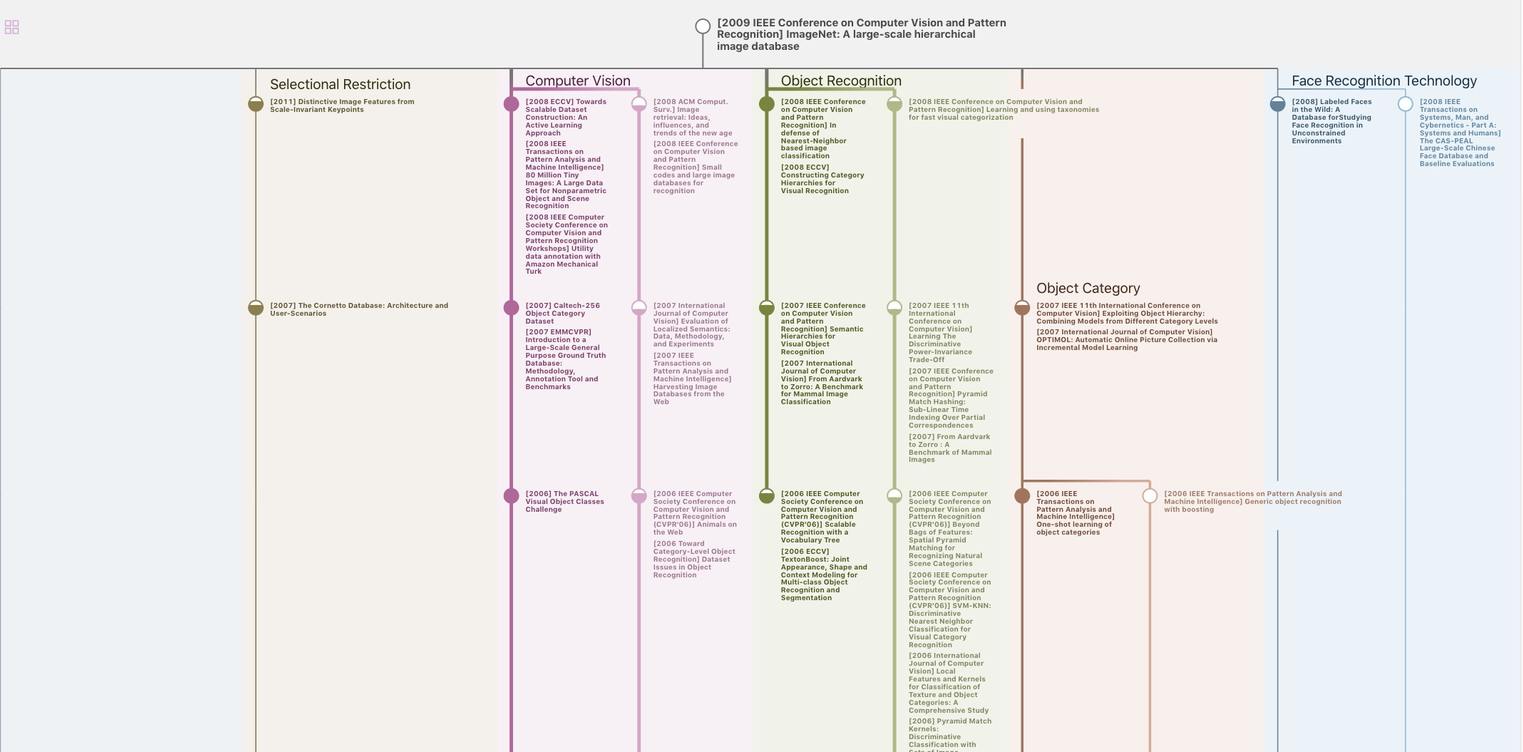
生成溯源树,研究论文发展脉络
Chat Paper
正在生成论文摘要