Efficient Probabilistic Prediction and Uncertainty Quantification of Tropical Cyclone-driven Storm Tides and Inundation
Artificial Intelligence for the Earth Systems(2023)
摘要
Abstract This study proposes and assesses a methodology to obtain high-quality probabilistic predictions and uncertainty information of near-landfall tropical cyclone(TC)-driven storm tide and inundation with limited time and resources. Forecasts of TC track, intensity, and size are perturbed according to quasi-random Korobov sequences of historical forecast errors with assumed Gaussian and uniform statistical distributions. These perturbations are run in an ensemble of hydrodynamic storm tide model simulations. The resulting set of maximum water surface elevations are dimensionality reduced using Karhunen-Lo`eve expansions and then used as a training set to develop a Polynomial Chaos (PC) surrogate model from which global sensitivities and probabilistic predictions can be extracted. The maximum water surface elevation is extrapolated over dry points incorporating energy head loss with distance to properly train the surrogate for predicting inundation. We find that the surrogate constructed with 3rd order PCs using Elastic Net penalized regression with Leave-One-Out cross-validation provides the most robust fit across training and test sets. Probabilistic predictions of maximum water surface elevation and inundation area by the surrogate model at 48-hour lead time for three past U.S. landfalling hurricanes (Irma 2017, Florence 2018, and Laura 2020) are found to be reliable when compared to best-track hindcast simulation results, even when trained with as few as 19 samples. The maximum water surface elevation is most sensitive to perpendicular track-offset errors for all three storms. Laura is also highly sensitive to storm size and has the least reliable prediction.
更多查看译文
关键词
storm tides,efficient probabilistic prediction,tropical cyclone–driven,uncertainty quantification
AI 理解论文
溯源树
样例
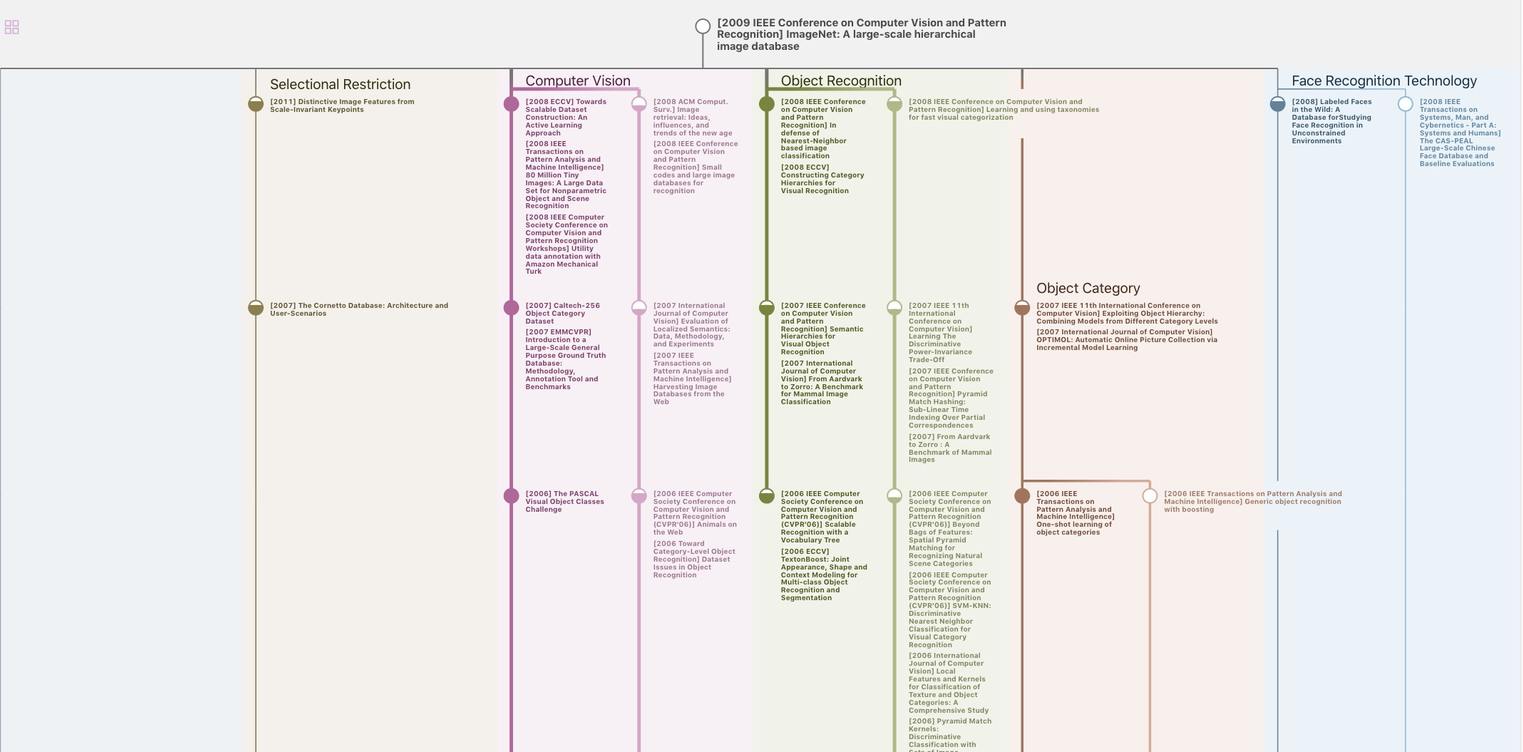
生成溯源树,研究论文发展脉络
Chat Paper
正在生成论文摘要