Efficient probabilistic storm surge estimation through adaptive importance sampling across storm advisories
Coastal Engineering(2023)
摘要
During landfalling tropical storms, probabilistic predictions of the storm surge constitute important products for guiding emergency response/preparedness decisions. The probabilistic formulation for these predictions is established by considering forecast errors for the intensity, size, cross- and along-track variability of the National Hurricane Center (NHC) advisories. These errors define ultimately the vector of uncertain storm features, with corresponding probability distributions chosen based on historical data. Propagation of the uncertainty in these features, serving as input to a numerical model for predicting storm surge, provides the desired statistical products, for example, the water level corresponding to a specific exceedance probability. This estimation is repeated whenever the NHC updates the storm advisory. Monte Carlo (MC) simulation is considered here as the numerical tool for facilitating this uncertainty propagation. Specifically, the implementation of adaptive importance sampling (IS) across the storm advisories is examined to improve MC computational efficiency, with objectives to attain better accuracy estimates or accommodate predictions using a smaller number of storm surge simulations. IS achieves this objective by introducing a proposal density (IS density) to choose a storm ensemble with higher contribution to the examined probabilistic product(s). In the proposed implementation, storm surge simulation results from the current advisory are leveraged to select the IS density to use for the next advisory, establishing an IS workflow that involves minimal additional computational burden, since readily available information is utilized. The requirement to estimate the storm surge across a large geographic domain, leading to the definition of a large number of quantities of interests (QoIs), poses a significant challenge in this case, since these quantities typically represent competing IS choices. Different schemes are discussed to establish a compromising solution, with emphasis on the use of principal component analysis to reduce the computational burden for the IS density selection. Alternative IS formulations are examined, and an adaptive selection of the IS characteristics is discussed, utilizing a novel global sensitivity analysis scheme and an efficient estimation of the anticipated IS accuracy. In order to guarantee robustness, since the IS density is established using limited information (i.e, limited number of simulations) involving a large number of QoIs, and, more importantly, it is chosen based on the information of the current advisory but is implemented in the next one, a defensive IS scheme is introduced. Validation considering different historical storms demonstrates robustness and improvements in accuracy, accomplished by the proposed sharing of information across advisories through an adaptive IS formulation. Extensions to accommodate a quasi-Monte Carlo implementation are also discussed.
更多查看译文
关键词
Landfalling storms,Storm forecast errors,Probabilistic surge estimation,Monte Carlo simulation,Adaptive importance sampling,High-dimensional output
AI 理解论文
溯源树
样例
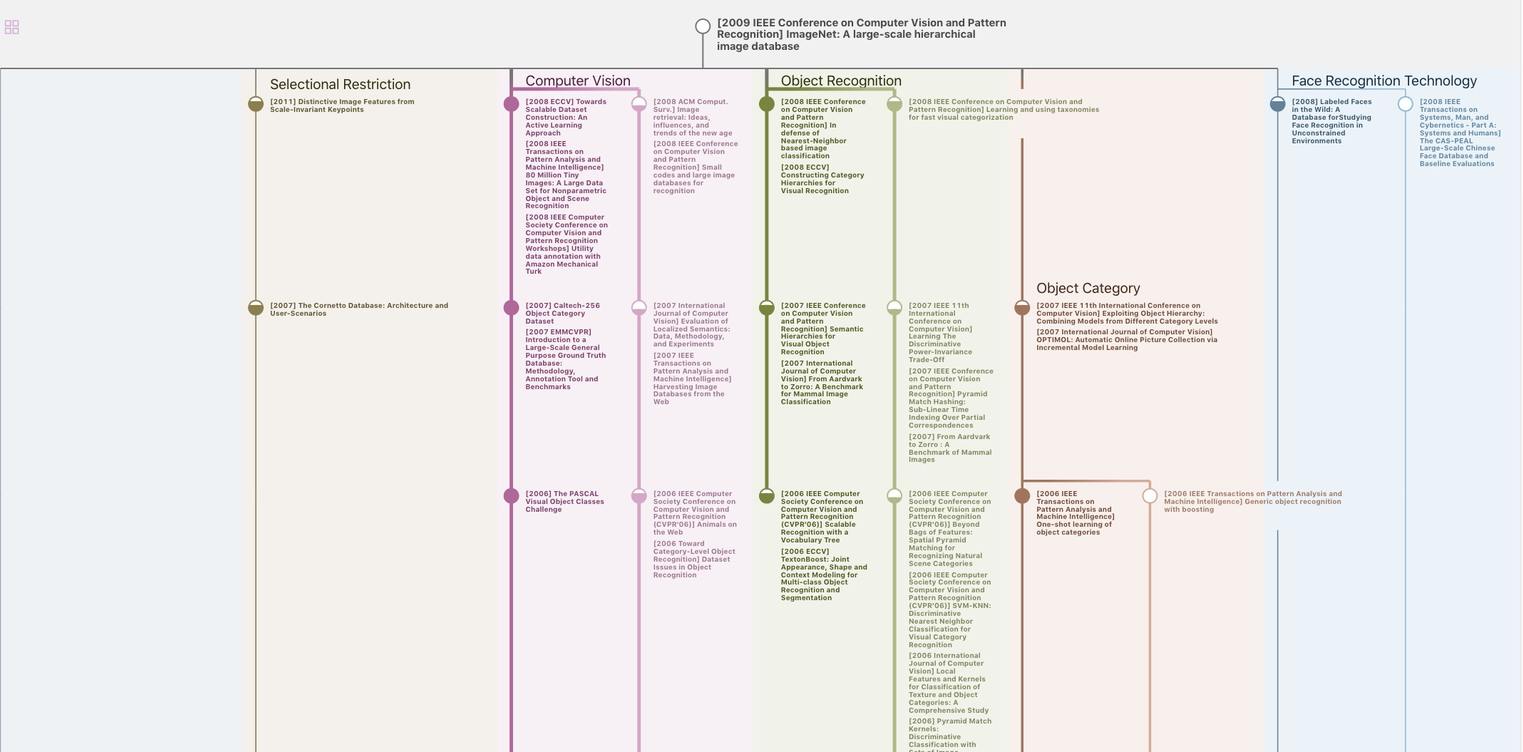
生成溯源树,研究论文发展脉络
Chat Paper
正在生成论文摘要