Climate downscaling for regional models with a neural network: A Hawaiian example
Progress in Oceanography(2023)
摘要
Global ocean/atmosphere circulation models (GCMs) allow us to better understand and predict the behavior of the ocean and atmosphere at large spatial and temporal scales; however, they may be unsuited for regional studies due to their low spatial resolution. We have developed a method for downscaling GCMs data using an artificial neural network to reconstruct high spatial resolution regional fields. We use these downscaled fields to force a regional ocean model and examine the efficacy of the method and results. The new downscaling method combines the multivariate empirical orthogonal function (EOF) with a convolutional neural network. Utilizing an EOF decomposition of high-resolution observations and/or regional model output, we train the neural network so that it will produce high-resolution fields based on the low-resolution GCM input. We apply this method to downscale GCM near-surface wind vectors in the region of the Hawaiian Islands and force a regional ocean simulation with the downscaled winds. We compare this to a simulation directly forced by the GCM winds. The results show that the ocean simulation forced by the downscaled GCM winds demonstrates a significant improvement in capturing the ocean dynamics: increasing wind energy input from the shear regions around the islands, while decreasing it in the lee of islands. In addition, the downscaled wind transfers twice as much energy to the deep ocean as the GCM wind.
更多查看译文
关键词
climate,regional models,neural network
AI 理解论文
溯源树
样例
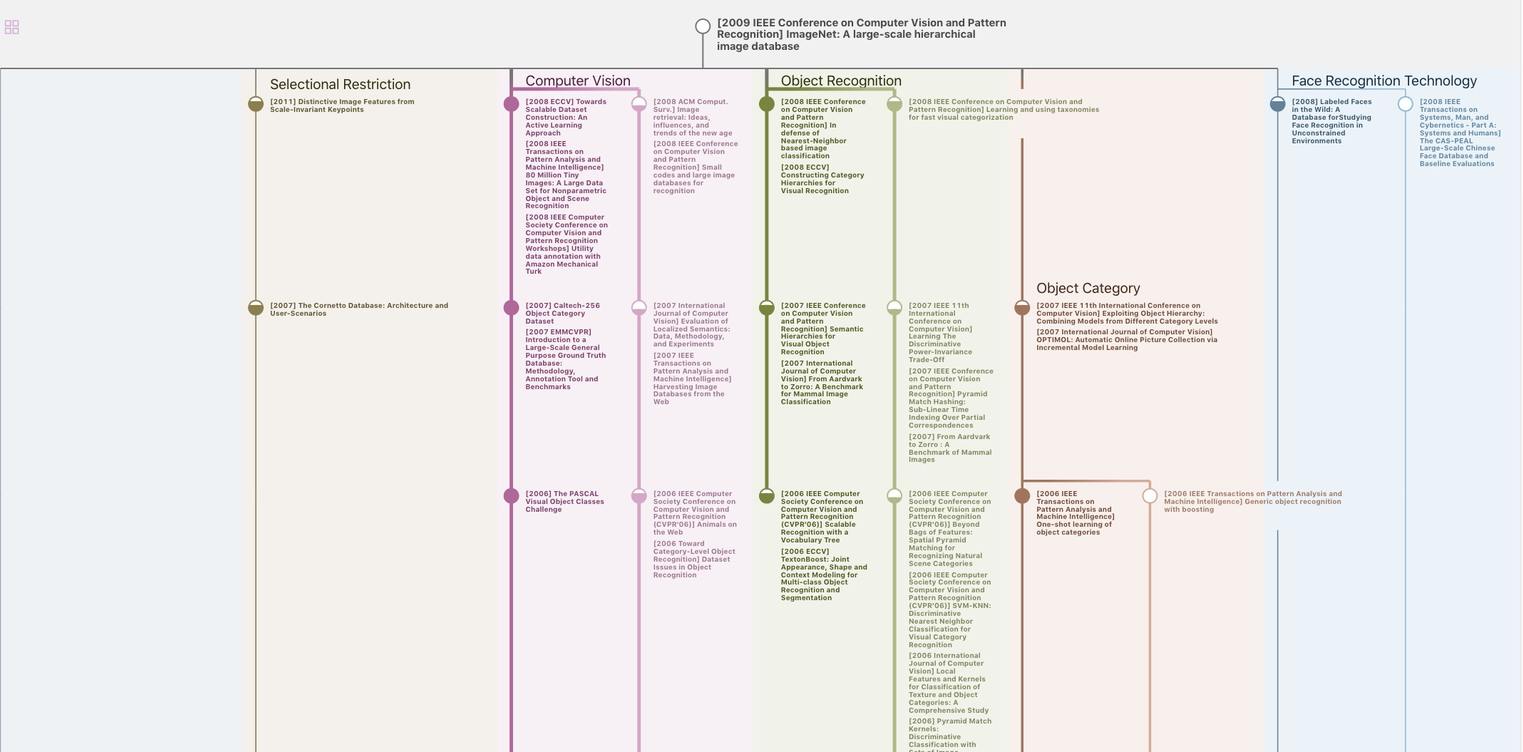
生成溯源树,研究论文发展脉络
Chat Paper
正在生成论文摘要