SleepSynth: Evaluating the use of Synthetic Data in Health Digital Twins.
ICDH(2023)
摘要
Health Digital Twins (HDTs) are virtual replicas of a patients physical/actual data. The major setbacks for applying Machine Learning (ML) in HDTs are the lack of availability of patients data due to privacy concerns and Artificial Intelligence (AI) bias. Given these shortcomings, synthetic data has been leveraged to solve privacy issues and increase diversity in datasets. In this paper, we evaluate four synthetic data generation models namely, Gaussian Copula, Conditional Tabular Generative Adversarial Network (CTGAN), CopulaGAN, and Tabular Variational Autoencoder (TVAE) which are used to generate synthetic data for actual sleep data retrieved from a wearable device. Gaussian Copula performed best in capturing the correlation between the variables with the real data with a quality score of approximately 96%. Additionally, we evaluate the efficacy of the synthetic generation models by training five well-known ML models on the generated synthetic data. Our experimental results show that the ML models trained on the synthetic data achieve an MAE (Mean Absolute Error) of less than 10% in the prediction of sleep quality score. The results from this work indicate that synthetic data could be used for ML tasks while preserving the privacy of data subjects.
更多查看译文
关键词
Digital Twin,Privacy,Synthetic Data,Wearable Data,Machine Learning,Copulas,Generative Adversarial Network (GAN)
AI 理解论文
溯源树
样例
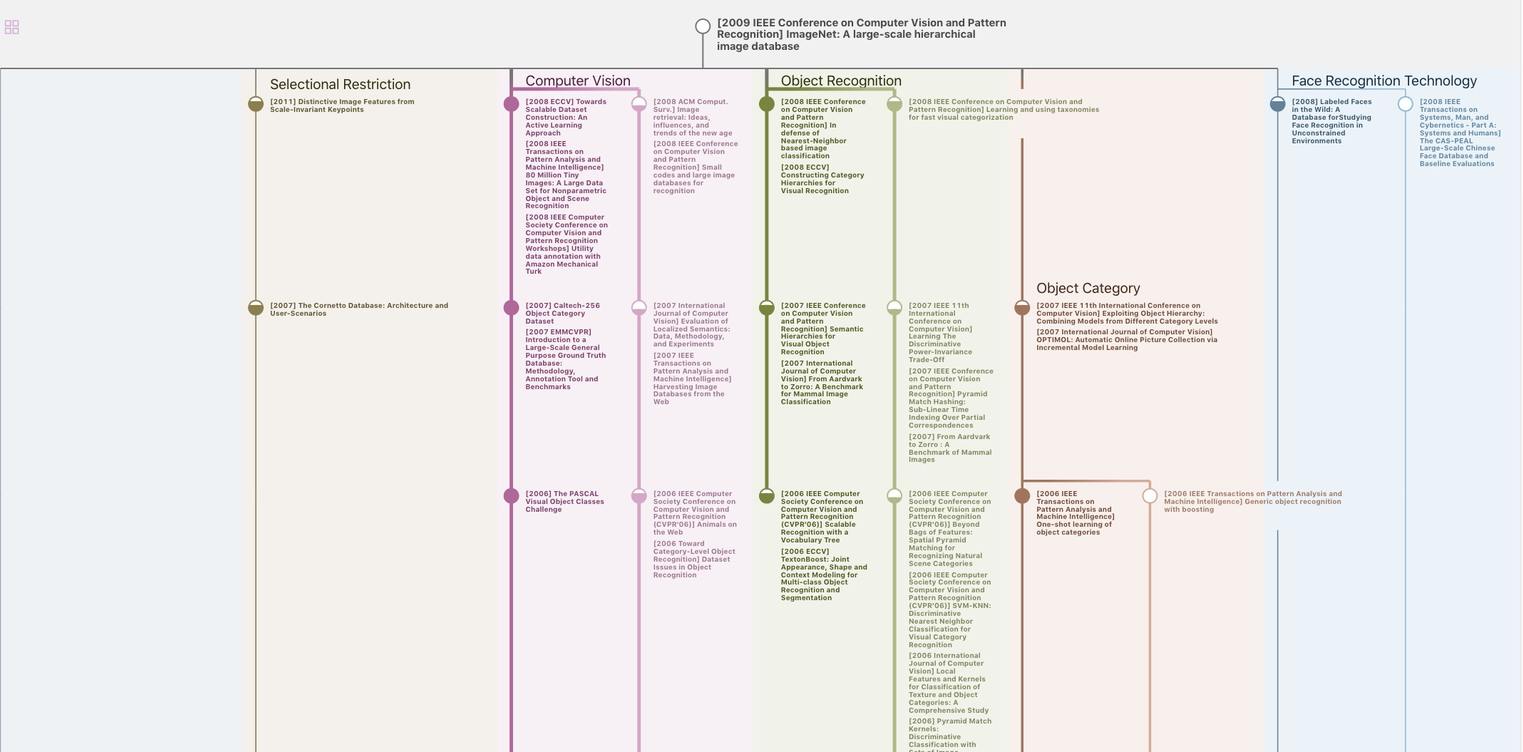
生成溯源树,研究论文发展脉络
Chat Paper
正在生成论文摘要