Spatially constrained atmosphere and surface retrieval for imaging spectroscopy
REMOTE SENSING OF ENVIRONMENT(2024)
摘要
Imaging spectrometers provide important Earth surface data for terrestrial and aquatic ecology, hydrology, geology through retrieved surface reflectance spectra. Surface reflectance is generally recovered from measured radiance through model inversions of the surface and atmospheric system. Most retrieval approaches treat all pixels independently and ignore spatial correlations in the atmosphere for neighboring pixels, resulting in surface-related biases in the retrieved surface and atmospheric parameters. In this work, we present a mathematical framework to more accurately retrieve surface reflectance and atmospheric parameters by leveraging the expected spatial smoothness of the atmosphere. This framework retrieves the full spatial- spectral reflectance data cube in a computationally efficient manner and is to our knowledge the first approach capable of accurately finding this global solution for large imaging spectroscopy datasets. We implement this mathematical framework in an example proposed algorithm which we call spatially constrained optimal estimation (SCOE). We show that SCOE improves the overall surface reflectance retrieval error, reduces surface -related biases in the retrieved surface reflectance and atmospheric parameters, and is more robust to noise than a traditional pixel-by-pixel approach and an operational empirical line approach in simulated and experimental results. This reduction in surface reflectance error will impact science data products across application areas, making imaging spectroscopy algorithms more robust for global acquisitions.
更多查看译文
关键词
Imaging spectroscopy,VSWIR,Retrieval algorithms,Computational imaging,Remote sensing,Earth science
AI 理解论文
溯源树
样例
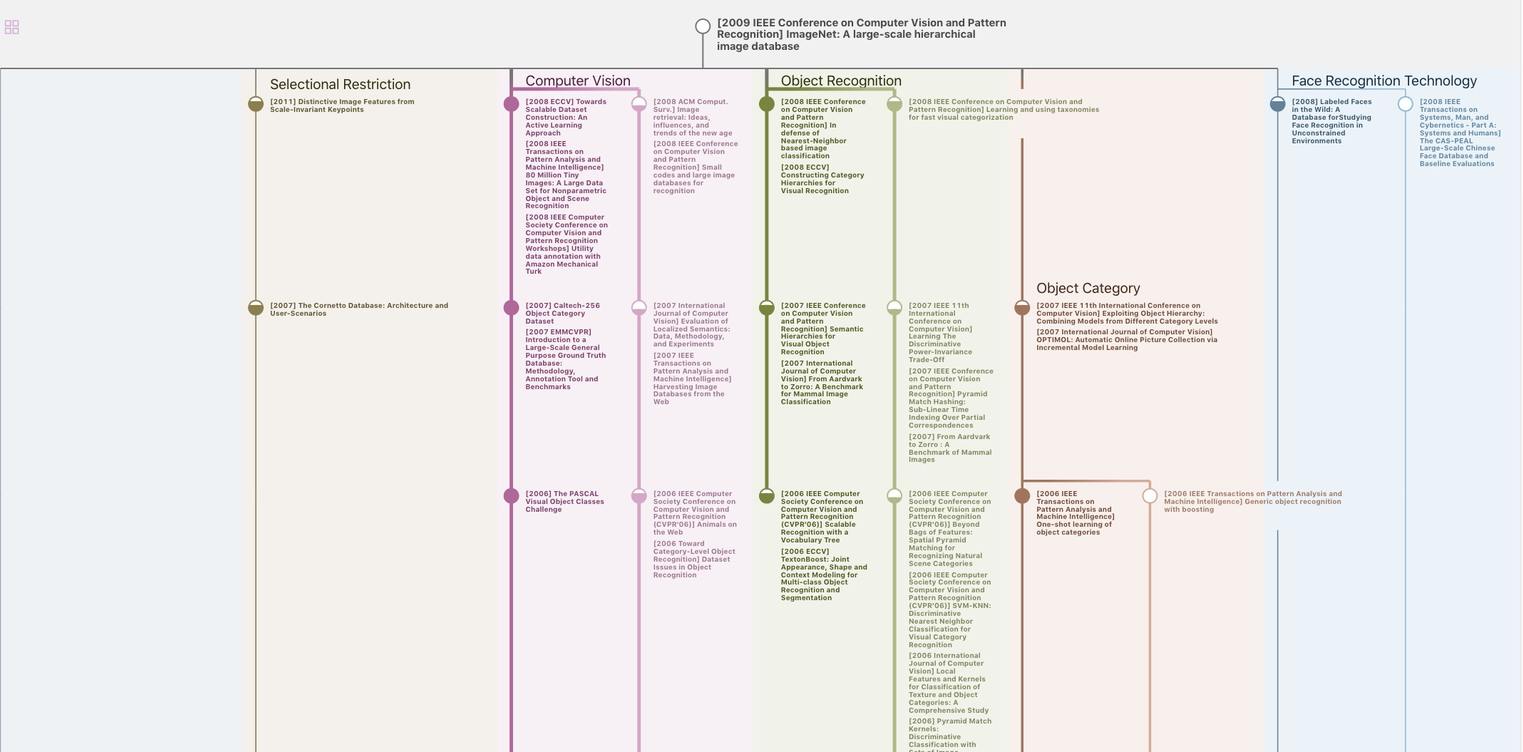
生成溯源树,研究论文发展脉络
Chat Paper
正在生成论文摘要