Machine Learning-Aided Rapid Estimation of Multilevel Capacity of Flexure-Identified Circular Concrete Bridge Columns with Corroded Reinforcement
JOURNAL OF STRUCTURAL ENGINEERING(2024)
摘要
Corrosion has been recognized as one of the predominant causes of degradation in aging RC bridge columns. This problem may significantly affect flexural and shear capacities and even alter failure modes of corroded columns. Traditional physics-based simulations used to determine the deteriorating capacity are time-consuming and may experience convergence problems, which hinders rapid and efficient fragility analyses of region-scale bridge infrastructure for the facilitation of regional risk assessment. To address the issue, this study leveraged machine learning techniques to identify column failure modes (flexure or shear-associated), followed by estimating the capacities of corroded RC columns with flexure failure, because flexural failure was found to be dominant among the considered large parameter spaces of corroded RC columns. To create the database, a finite-element model of RC columns considering bond-slip effects and deteriorating material strengths due to reinforcement corrosion was developed and validated experimentally to capture the flexural and shear behavior, and pushover analyses were preformed to examine the failure mode and quantify multilevel capacities of corroded columns. The performance of two machine learning algorithms (i.e., logistic regression and support vector machine) was evaluated for failure mode identification, and four additional algorithms (i.e., multiple linear regression, modified multiple linear regression, artificial neural network, and gradient boosting decision tree) were examined for the multilevel drift ratio capacity prediction of the flexure-identified corroded RC columns. The results show that the support vector machine and artificial neural network outperformed the other algorithms in failure mode identification and the drift ratio capacity prediction, respectively. In addition, the aspect ratio of columns was identified as the most significant feature for the drift ratio capacity of corroded RC columns, followed by reinforcement corrosion levels.
更多查看译文
关键词
Bridge,Corrosion,RC columns,Failure mode identification,Seismic capacity,Machine learning
AI 理解论文
溯源树
样例
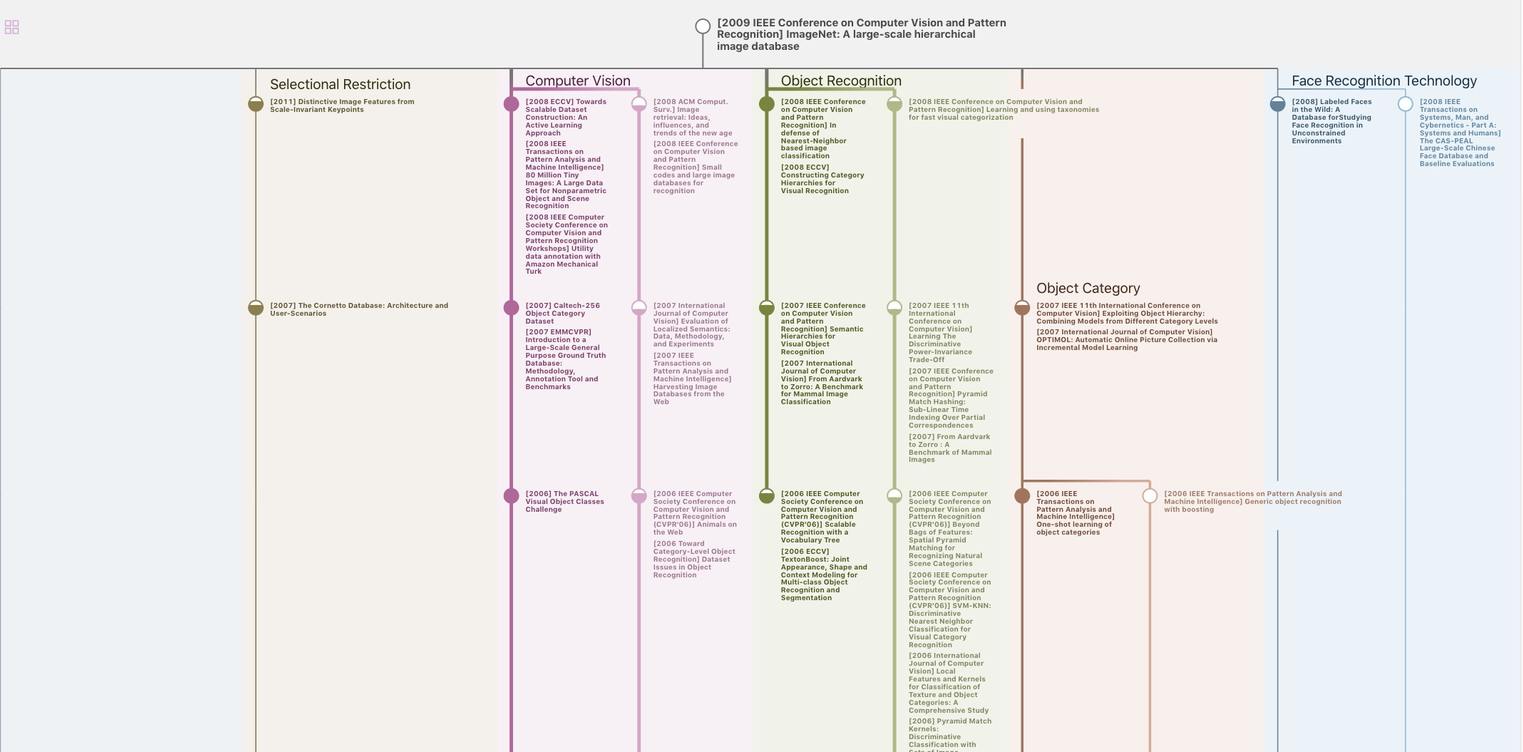
生成溯源树,研究论文发展脉络
Chat Paper
正在生成论文摘要