Generalizability of AI-based Emulators for CFD Lagrangian methods 
crossref(2024)
摘要
The combination of Computational Fluid Dynamics (CFD) and Artificial Intelligence (AI) enables to expand the scope of fluid modeling and improve simulation performance. Eulerian methods have already been extensively integrated with AI, providing high-fidelity and reliable results (e.g., weather prediction); the application of AI to Lagrangian methods remains less consolidated. Smoothed Particle Hydrodynamics (SPH) is a Lagrangian mesh-less CFD numerical method with well-established advantages for the simulation of highly dynamic free-surface complex fluids. However, reliable SPH simulations require long execution times and large computational resources. This downside can be resolved using emulators, which can reproduce the dynamics of a physical system, speeding-up the simulations. In detail, an emulator is a model in which AI algorithms join or replace the equation-based mathematical representation of physics. Thus, the emulator learns from CFD simulations the behavior of the CFD reference model and reproduces it to solve fluid dynamics problems in shorter times. For the emulator to be trusted, it is important to assess the ability to generalize and correctly predict the behavior of the fluid in conditions that have not been presented during training phase. Here, we present the generalizability of an AI-based emulator for SPH, utilizing an Artificial Neural Network (ANN) to estimate the hydrodynamic forces between SPH particles. We demonstrate the capability of the emulator to reproduce particle interaction quantities, as opposed to their specific spatial configuration. Therefore, when a different problem is treated, as long as the variables values are in a range with physics meaning presented during training, the emulator will be capable to produce physically meaningful results. We discuss the salient points of designing this emulator, such as the choice of the AI model, i.e., the ANN, and the choice of the features. We show applications to different test cases, highlighting the emulator ability to generalize for problems with varying levels of complexity, and for changes in the model resolution.
更多查看译文
AI 理解论文
溯源树
样例
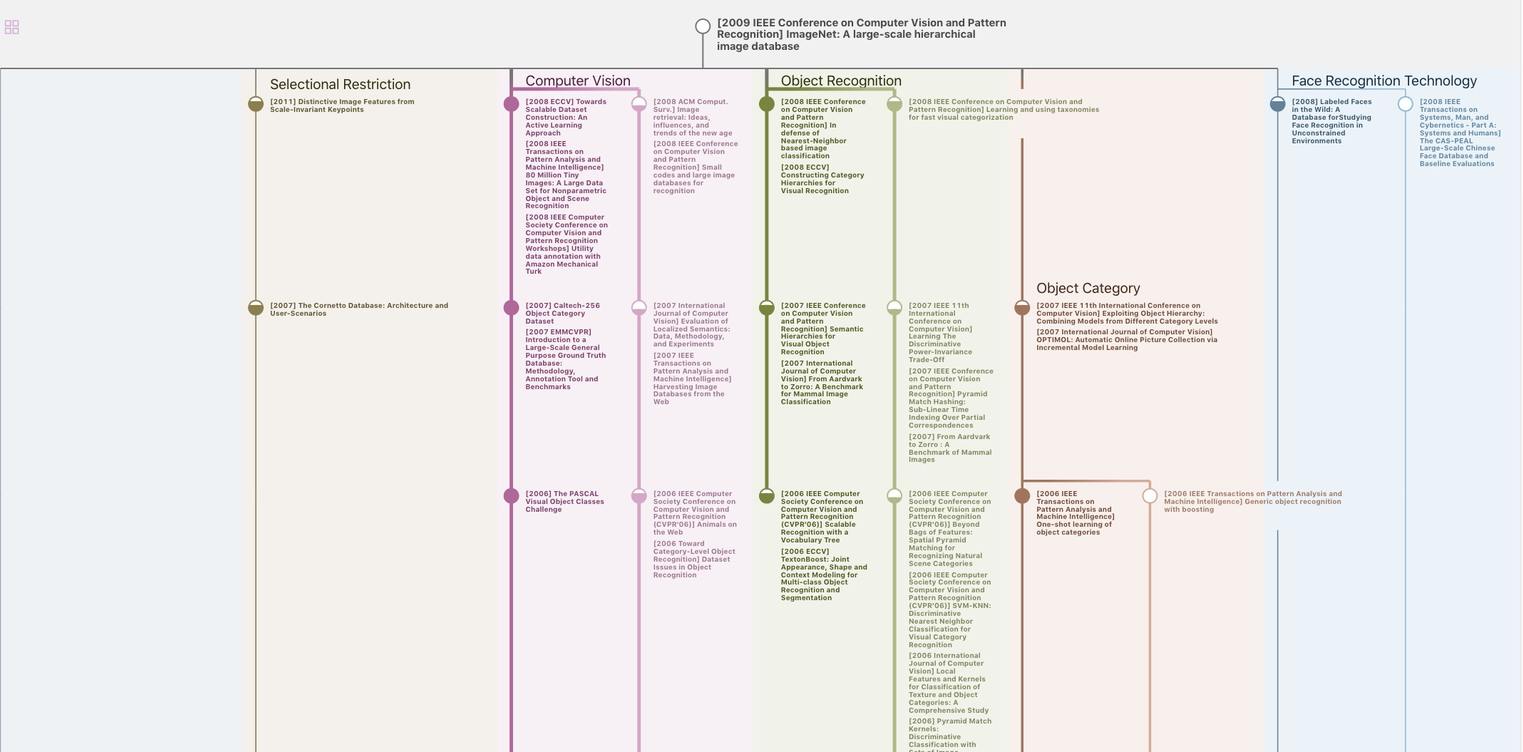
生成溯源树,研究论文发展脉络
Chat Paper
正在生成论文摘要