Ultra Low-Cost Two-Stage Multimodal System for Non-Normative Behavior Detection
arxiv(2024)
摘要
The online community has increasingly been inundated by a toxic wave of
harmful comments. In response to this growing challenge, we introduce a
two-stage ultra-low-cost multimodal harmful behavior detection method designed
to identify harmful comments and images with high precision and recall rates.
We first utilize the CLIP-ViT model to transform tweets and images into
embeddings, effectively capturing the intricate interplay of semantic meaning
and subtle contextual clues within texts and images. Then in the second stage,
the system feeds these embeddings into a conventional machine learning
classifier like SVM or logistic regression, enabling the system to be trained
rapidly and to perform inference at an ultra-low cost. By converting tweets
into rich multimodal embeddings through the CLIP-ViT model and utilizing them
to train conventional machine learning classifiers, our system is not only
capable of detecting harmful textual information with near-perfect performance,
achieving precision and recall rates above 99% but also demonstrates the
ability to zero-shot harmful images without additional training, thanks to its
multimodal embedding input. This capability empowers our system to identify
unseen harmful images without requiring extensive and costly image datasets.
Additionally, our system quickly adapts to new harmful content; if a new
harmful content pattern is identified, we can fine-tune the classifier with the
corresponding tweets' embeddings to promptly update the system. This makes it
well suited to addressing the ever-evolving nature of online harmfulness,
providing online communities with a robust, generalizable, and cost-effective
tool to safeguard their communities.
更多查看译文
AI 理解论文
溯源树
样例
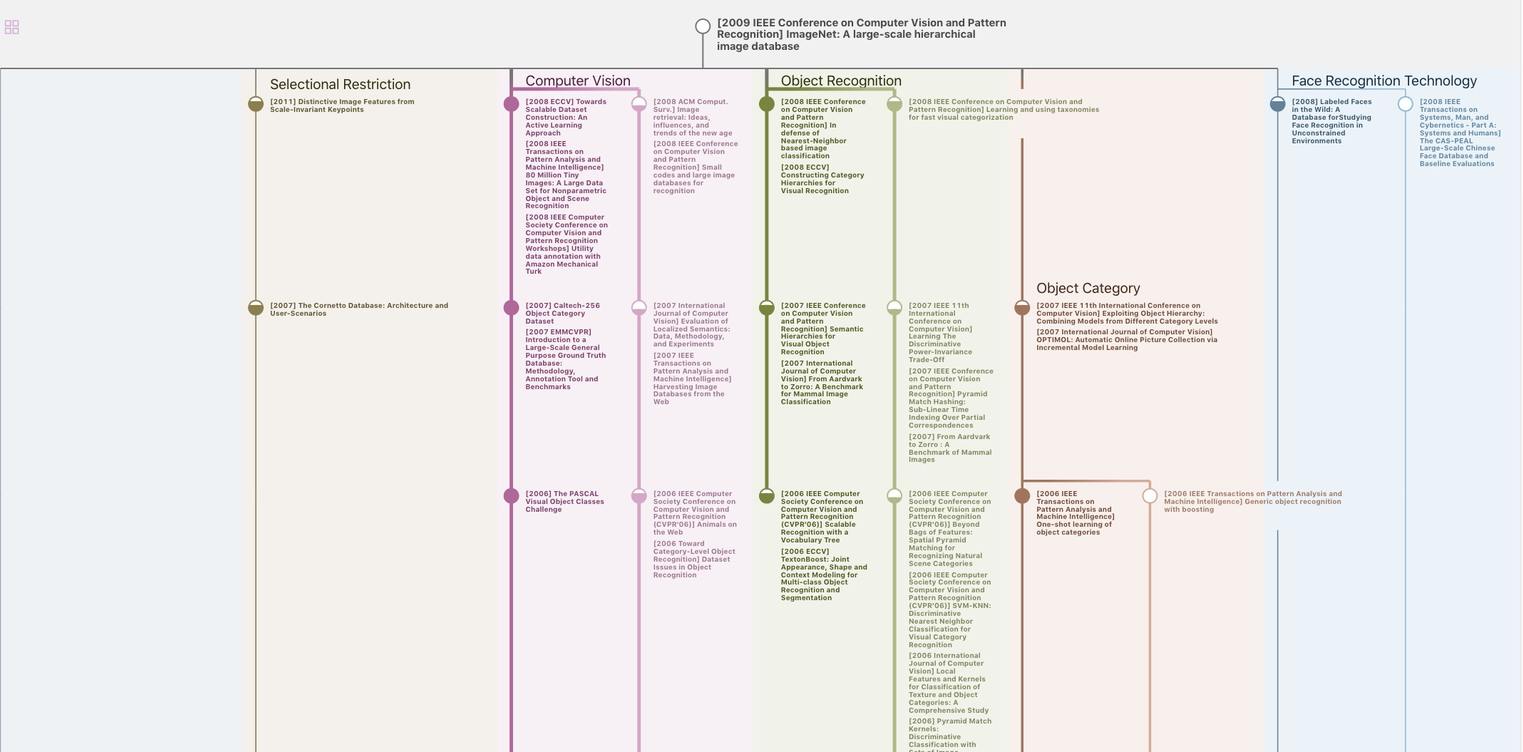
生成溯源树,研究论文发展脉络
Chat Paper
正在生成论文摘要