Federated Partial Label Learning with Local-Adaptive Augmentation and Regularization
AAAI 2024(2024)
摘要
Partial label learning (PLL) expands the applicability of supervised machine learning models by enabling effective learning from weakly annotated overcomplete labels. Existing PLL methods however focus on the standard centralized learning scenarios. In this paper, we expand PLL into the distributed computation setting by formalizing a new learning scenario named as federated partial label learning (FedPLL), where the training data with partial labels are distributed across multiple local clients with privacy constraints. To address this challenging problem, we propose a novel Federated PLL method with Local-Adaptive Augmentation and Regularization (FedPLL-LAAR). In addition to alleviating the partial label noise with moving-average label disambiguation, the proposed method performs MixUp-based local-adaptive data augmentation to mitigate the challenge posed by insufficient and imprecisely annotated local data, and dynamically incorporates the guidance of global model to minimize client drift through adaptive gradient alignment regularization between the global and local models. Extensive experiments conducted on multiple datasets under the FedPLL setting demonstrate the effectiveness of the proposed FedPLL-LAAR method for federated partial label learning.
更多查看译文
关键词
ML: Distributed Machine Learning & Federated Learning,ML: Classification and Regression,ML: Deep Learning Algorithms
AI 理解论文
溯源树
样例
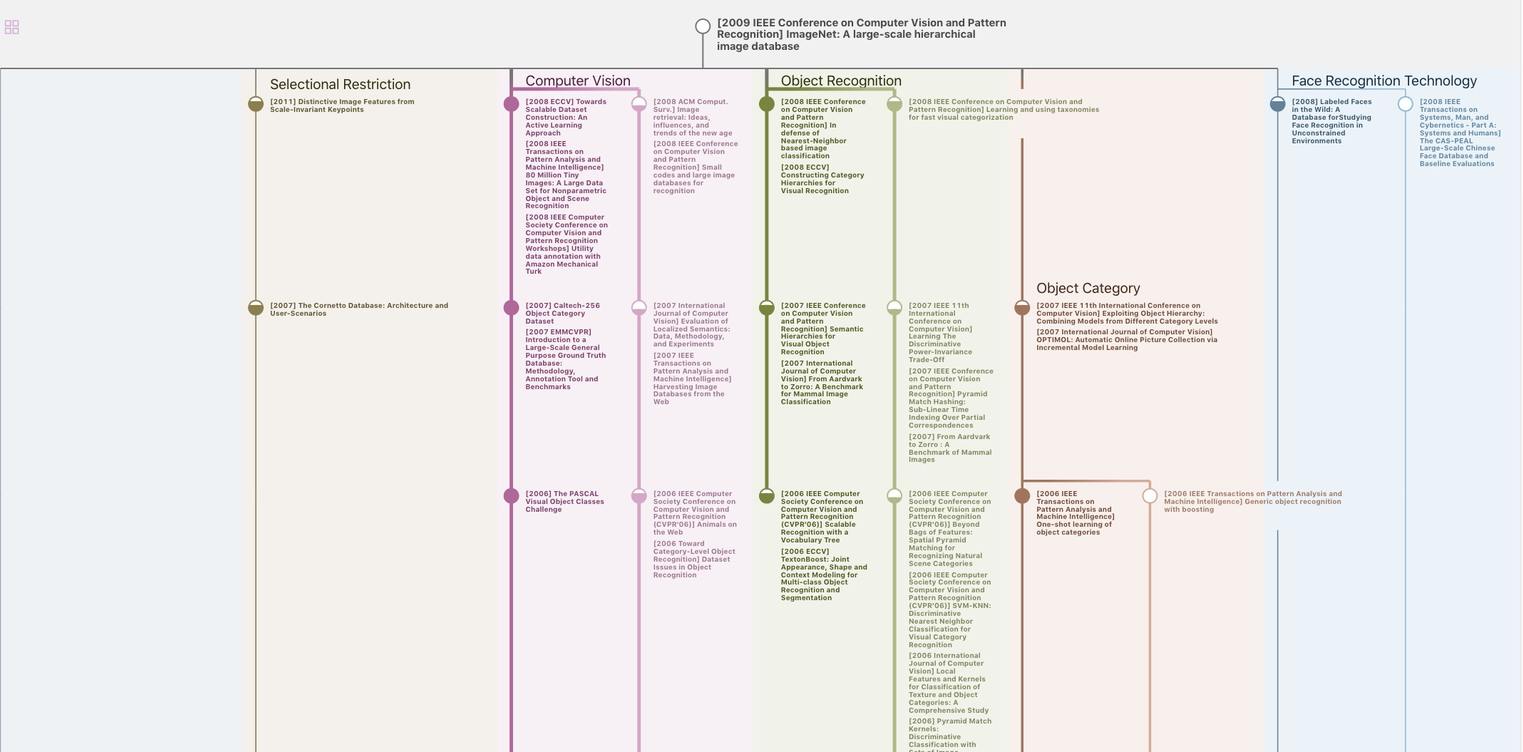
生成溯源树,研究论文发展脉络
Chat Paper
正在生成论文摘要