Machine Learning-Based Wave Model With High Spatial Resolution in Chesapeake Bay
EARTH AND SPACE SCIENCE(2024)
摘要
A high-resolution wave model is crucial for accurate modeling of sediment and organic material transports, but its computational costs hinder direct coupling to an ecosystem model. We developed a machine learning model using long short-term memory to simulate large-scale, high-resolution waves. Trained with numerical wave model (NWM) outputs and wind data from nine locations, our model successfully replicates NWM results for daily mean significant wave height and period in Chesapeake Bay with identical spatial resolution. Compared to the NWM, the data-driven model has root-mean-square errors below 6 cm for daily mean significant wave height and 1 s for the wave period in the bay. It demonstrates excellent model skills and can accurately forecast daily mean significant wave height and period at NOAA wave stations comparable to NWMs. Using minimal wind data and having a short runtime, our data-driven model shows promise as an alternative for wave forecasting and coupling with sediment and ecological models. Accurately modeling sediment and organic transport necessitates the use of a high-resolution numerical wave model (NWM). However, the computational expenses of running an NWM within an ecosystem model pose significant challenges. We conducted a study using a machine learning approach based on long short-term memory to replicate the results of an NWM. The data-driven wave model (DWM) was trained with the NWM outputs and wind data from nine locations. The trained DWM can replicate the NWM results of daily mean significant wave height and period in Chesapeake Bay with identical spatial resolution and the same predictive skill with a root-mean-square error of less than 6 cm and 1 s, respectively for the model domain. The model successfully forecasted daily mean significant wave height and period at NOAA wave stations, showing great forward prediction capability. Notably, the DWM only relied on a minimal amount of wind data from selected locations and had a short runtime. These findings indicate that machine learning is promising as an alternative approach to NWM for wave forecasting. Moreover, it can serve as an alternative wave model and be coupled to ecological models for simulating sediment transport, light attenuation, and resuspension of organic materials. A machine learning-based wave model reproduces the numerical wave model (NWM) results at the same spatial resolution with good accuracy The data-driven model, trained on daily significant wave height and period using NWM outputs, has comparable wave prediction skill The fast-running data wave model, driven by wind data from nine locations, can forecast waves and couple with sediment and ecological models
更多查看译文
关键词
wave,machine learning,data-driven model,SCHISM,Chesapeake Bay
AI 理解论文
溯源树
样例
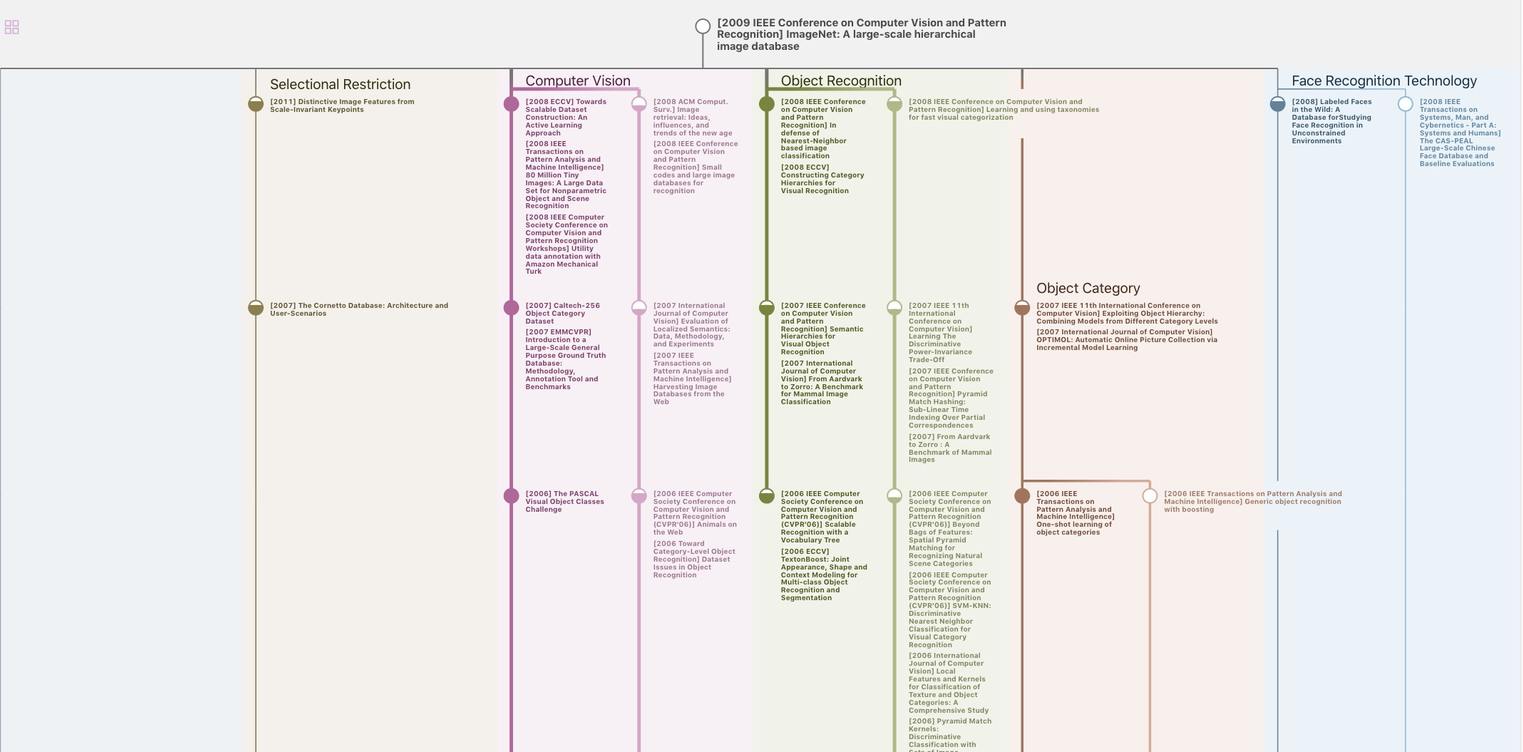
生成溯源树,研究论文发展脉络
Chat Paper
正在生成论文摘要