Learning-Based Resource Optimization In Ultra Reliable Low Latency Hetnets
2019 IEEE GLOBAL COMMUNICATIONS CONFERENCE (GLOBECOM)(2019)
摘要
In this paper, the problems of user offloading and resource optimization are jointly addressed to support ultra reliable and low latency communications (URLLC) in HetNets. In particular, a multi-tier network with a single macro base station (MBS) and multiple overlaid small cell base stations (SBSs) is considered that includes users with different latency and reliability constraints. Modeling the latency and reliability constraints of users with probabilistic guarantees, the joint problem of user offloading and resource allocation (JUR) in a URLLC setting is formulated as an optimization problem to minimize the cost of serving users for the MBS. In the considered scheme, SBSs bid to serve URLLC users under their coverage at a given price, and the MBS decides whether to serve each user locally or to offload it to one of the overlaid SBSs. Since the JUR optimization is NP-hard, we propose a low complexity learning based heuristic method (LHM) which includes a support vector machine-based user association model and a convex resource optimization (CRO) algorithm. To further reduce the delay, we propose an alternating direction method of multipliers (ADMM)-based solution to the CRO problem. Simulation results show that using LHM, the MBS significantly decreases the spectrum access delay for users (by similar to 93%) as compared to JUR, while also reducing its bandwidth and power costs in serving users (by similar to 33%) as compared to directly serving users without offloading.
更多查看译文
关键词
User association,resource optimization,user offloading,URLLC,HetNets
AI 理解论文
溯源树
样例
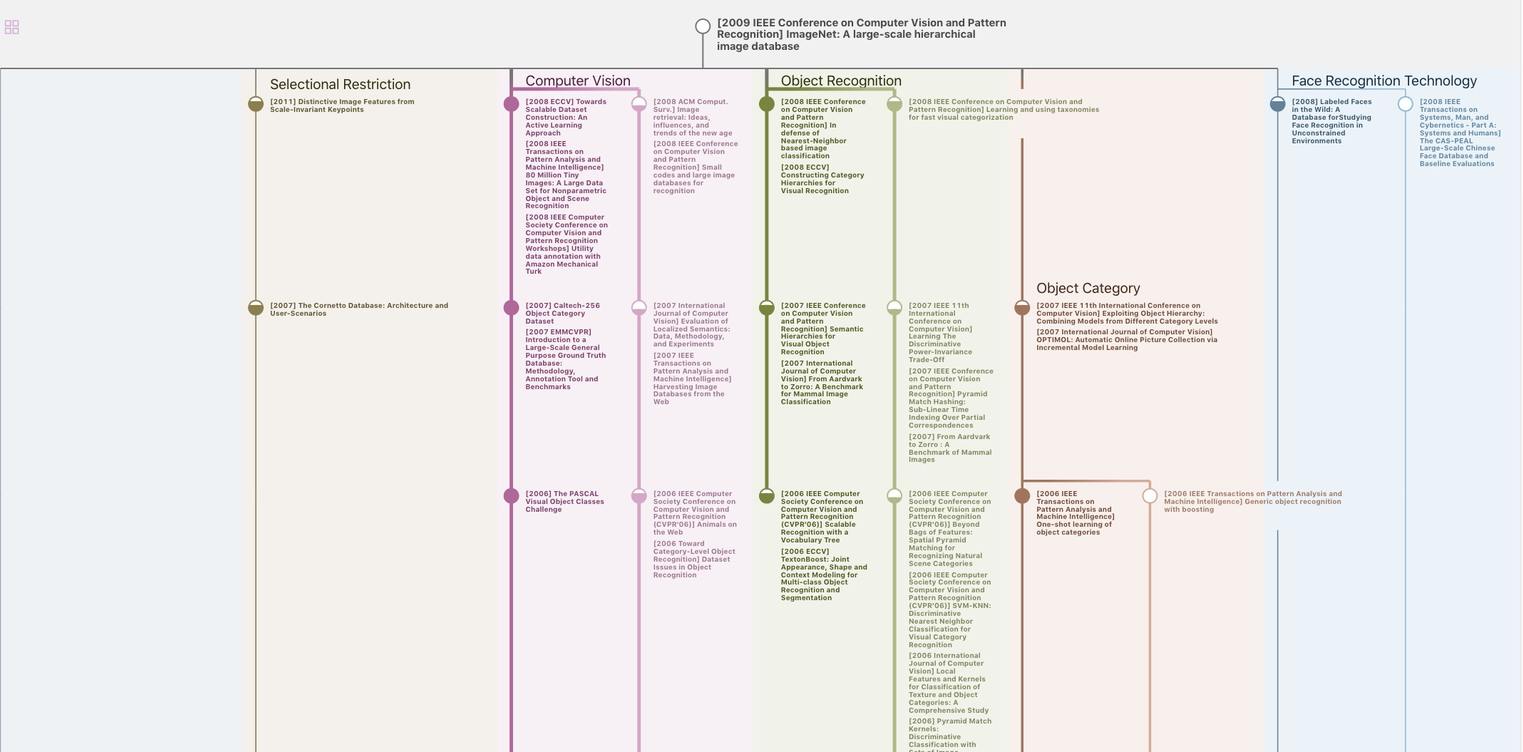
生成溯源树,研究论文发展脉络
Chat Paper
正在生成论文摘要